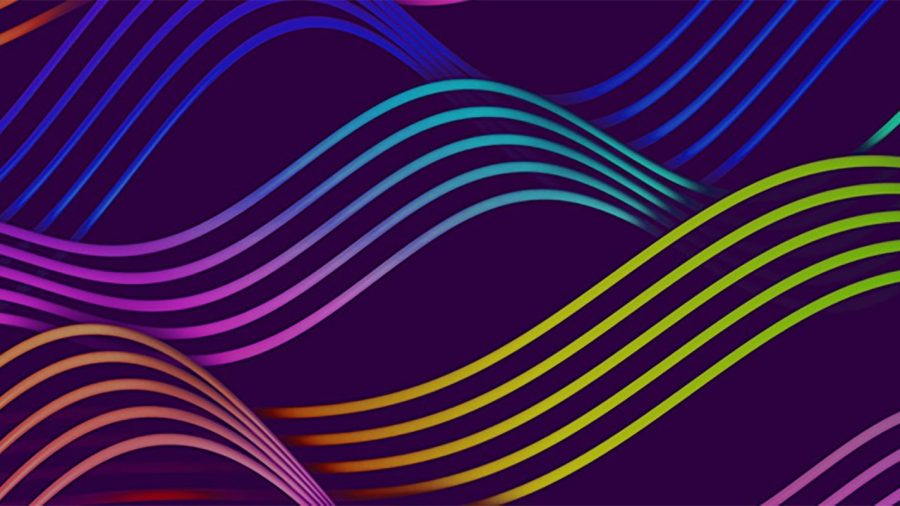
OpenAI has released MuseNet – a deep neural network that creates musical compositions with 10 different instruments. The neural network model is based on the general unsupervised method as the popular GPT-2 model.
GPT-2 is in fact, a large-scale transformer model which was trained to simply predict the next token in a sequence of data, regardless of the type of the data. Similarly, MuseNet was not explicitly trained to understand music or audio data, but instead, it tries to predict the next token in hundreds of music files.
Researchers from OpenAI explain that MuseNet is able to learn long-term structure in music because it uses the optimized kernels from Sparse Transformer models – developed recently by OpenAI as more efficient transformer models.
The MuseNet model was trained using a large number of MIDI music files provided by Classical Archives, BitMidi, and other online sources. The transformer model consisting of 72 layers and 24 attention heads is trained on sequential data trying to predict the next note in a sequence.
Researchers tried several approaches towards encoding the MIDI files into tokens that will allow the transformer model to capture long-term structure. According to them, the best encoding scheme was the one combining the pitch, volume, and instrument information into a single token. During the training phase, they also augmented the data by transposing notes, augmenting volume and timing and using mixup on the token embeddings space. Moreover, they introduced an “inner critic” score which is computed by the network by predicting whether a given sample is truly from the dataset or if it is one of the model’s own past generations.
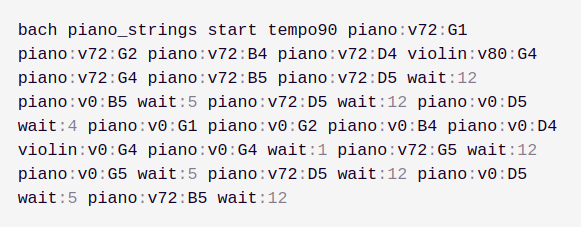
Sample compositions created with MuseNet are provided by OpenAI at their official blog. They also released a MuseNet-powered co-composer tool which will be available through May 12th.