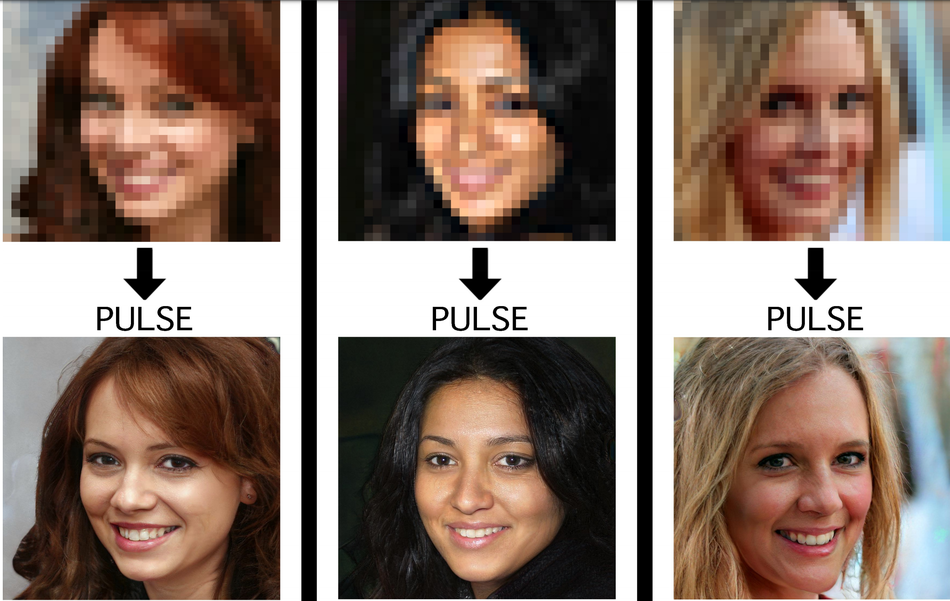
A group of researchers from Duke University has proposed a novel state-of-the-art method for image super-resolution that is able to generate realistic very high-resolution images from a low-resolution input image. The method, called PULSE, is able to learn how to perform photo upsampling in a completely self-supervised manner.
It works by leveraging the advances in Generative Adversarial Networks (GANs) to traverse the high-resolution natural image manifold and search for images that downscale to the input low-resolution image. Researchers employ several constraints in their search in order to reduce the search space and make sure that the generated output images are realistic. With this approach, they introduced a new paradigm for image super-resolution as well as a novel method for latent space exploration under high-dimensional Gaussian prior distributions.
PULSE was evaluated using several different types of experiments. Researchers used the popular Face StyleGAN for the purpose of solving the task of face hallucination. The experiments were done using the popular CelebA HQ dataset that contains high-resolution images of human faces. It was shown that PULSE can generate super-resolved images that are realistic and downscale accurately. The new method proved to be superior in both quantitative and qualitative ways over existing state-of-the-art methods.
The implementation of PULSE was open-sourced and is available on Github. The paper was published on arxiv.