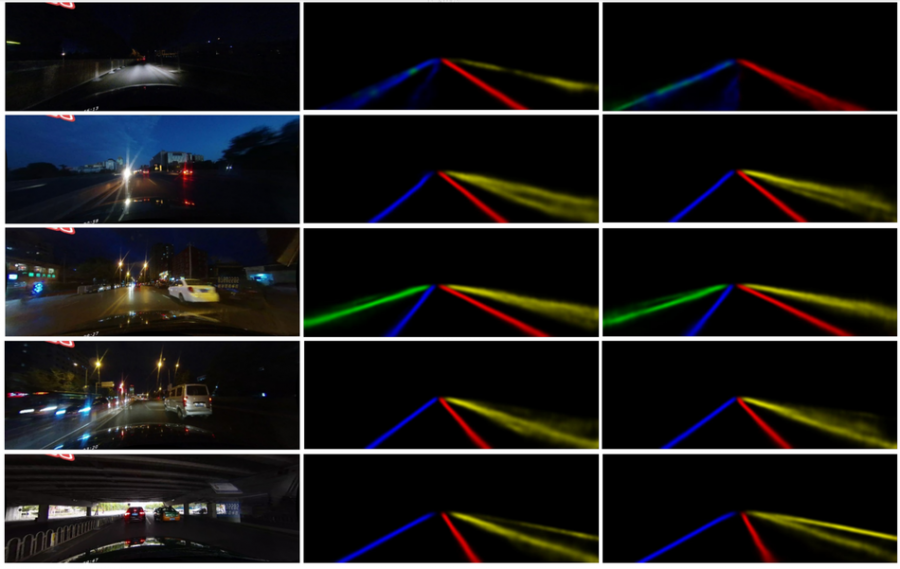
A group of researchers from the Intelligent Navigation Laboratory at Beijing Institute of Technology has proposed a novel method that uses GANs to detect lane markings in low-light conditions.
In their paper, “Lane Detection in Low-light Conditions Using an Efficient Data Enhancement: Light Conditions Style Transfer”, researchers present an interesting idea where they use a style-transfer model to generate images in low-light conditions and afterward train a more robust lane detection models.
Their novel method employs GANs or more specifically a CycleGAN architecture to perform data enhancement and it improves lane detection models’ adaptability without any additional annotations nor additional computation at inference time.
Researchers designed a novel GAN architecture for the task of generating low-light images which they named Better-CycleGAN. It comprises of two generator networks: one which transfers images from normal to low-light conditions and the other which does the opposite – from low-light to normal light conditions. The two discriminators are trained to discriminate between real and fake and they provide this value to the generators at training time.
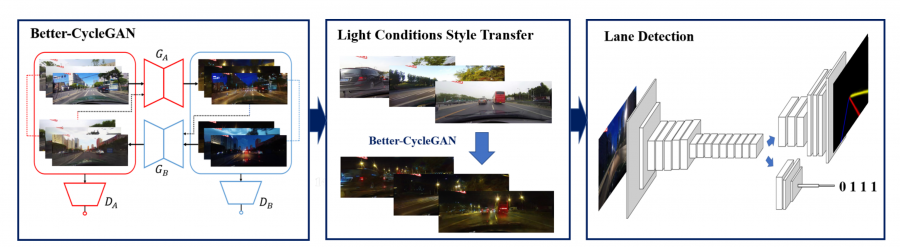
The generated images were used to train a baseline lane detection model which was chosen to be ERF-Net. The method was then evaluated on the popular CULane lane detection benchmark and the results showed that the method exhibits more robustness and adaptability to complex scenarios.
More in detail about the proposed method can be read in the paper published in arxiv. The implementation of the method will be publicly available.