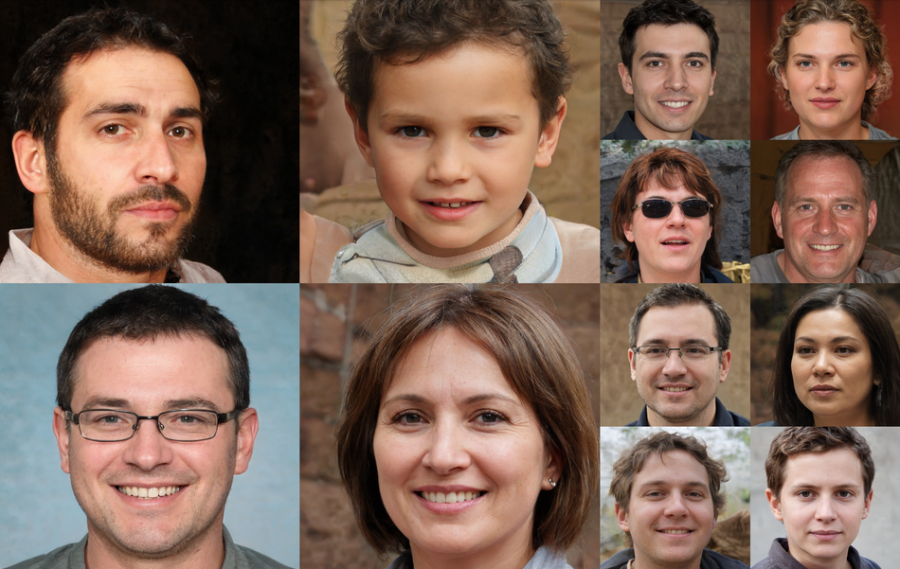
Researchers from NVIDIA have published an updated version of StyleGAN – the state-of-the-art image generation method based on Generative Adversarial Networks (GANs), which was also developed by a group of researchers at NVIDIA.
StyleGAN was known as the first generative model that is able to generate impressively photorealistic images and offers control over the style of the generated image. These results were achieved by introducing some radical changes to the generator network as part of the GAN architecture.
Now, researchers led by Tero Karras have published a paper where they analyze the capabilities of the original StyleGAN architecture and propose a new improved version – StyleGAN2. They propose several modifications both in the architecture and the training method of StyleGAN.
In particular, researchers redesigned the generator normalization, they revisited the progressive growing as the training stabilization and introduced a new regularization technique to improve conditional generation. The generator architecture was modified such that AdaIn layers were removed i.e adaptive instance normalization was replaced with a “demodulation” operation. After inspecting the effects of progressive growing as a procedure for training with large resolution images, researchers propose an alternative approach where training starts by focusing on low-resolution images and then progressively shifts focus to higher and higher resolutions but without changing the network topology.
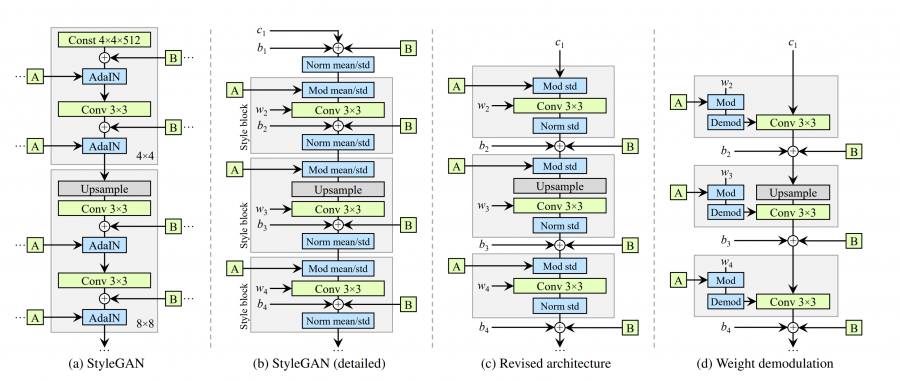
Researchers evaluated the proposed improvements using several datasets and showed that the new architecture redefines the state-of-the-art achievements in image generation. According to them, the method performs better than StyleGAN both in terms of distribution quality metrics as well as in perceived image quality.
More about StyleGAN2 can be read in the pre-print paper published on arxiv. The Tensorflow implementation of the method was open-sourced and it’s available on Github.