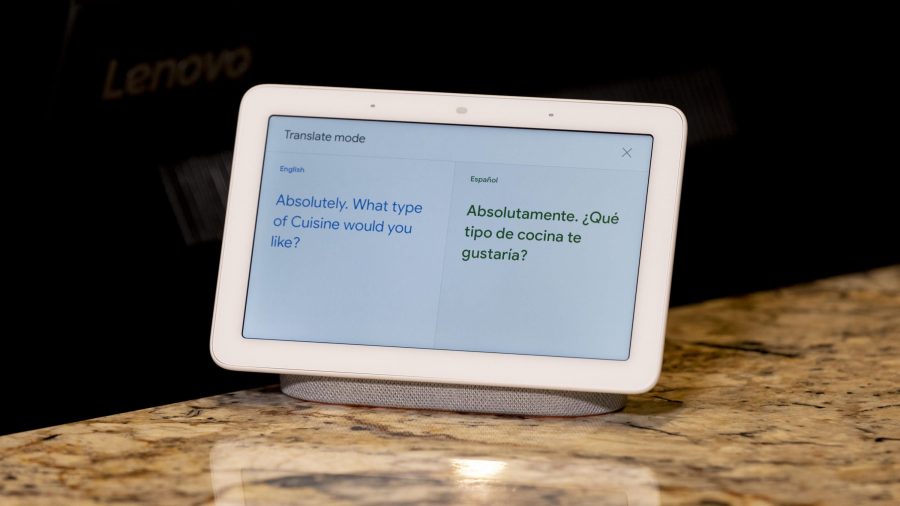
Researchers from Google AI have proposed a new speech-to-speech translation model and a new experimental system that shows faster inference and improved quality of the translation.
The new translation model is an attentive sequence-to-sequence model that does not rely on intermediate text representation. The end-to-end model proposed in “Direct speech-to-speech translation with a sequence-to-sequence model” does not have separate stages for dividing the task of translation but instead learns a direct mapping from one speech sequence to another.
It uses spectrograms as inputs and generates spectrograms of the translated content in an end-to-end manner. The model employs an encoder-decoder architecture and two separate and optional components: vocoder for transforming the output to waveform and a speaker encoder to encode speakers voice in the case it is used to maintain the same voice in the translated speech. Both of the additional components are trained separately.
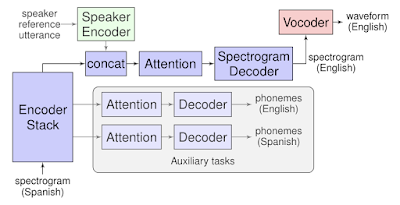
Researchers conducted extensive experiments to evaluate the proposed model and concluded that it is performing well and overcoming the problems that exist in multi-stage methods. They showed that the model is able to preserve the speaker’s voice in the translation.
Translatotron represents the first end-to-end model for translating speech from one language to another without intermediate text. More details about the model’s architecture and evaluation samples can be found in the official blog post. The paper was published as a pre-print paper on arxiv.