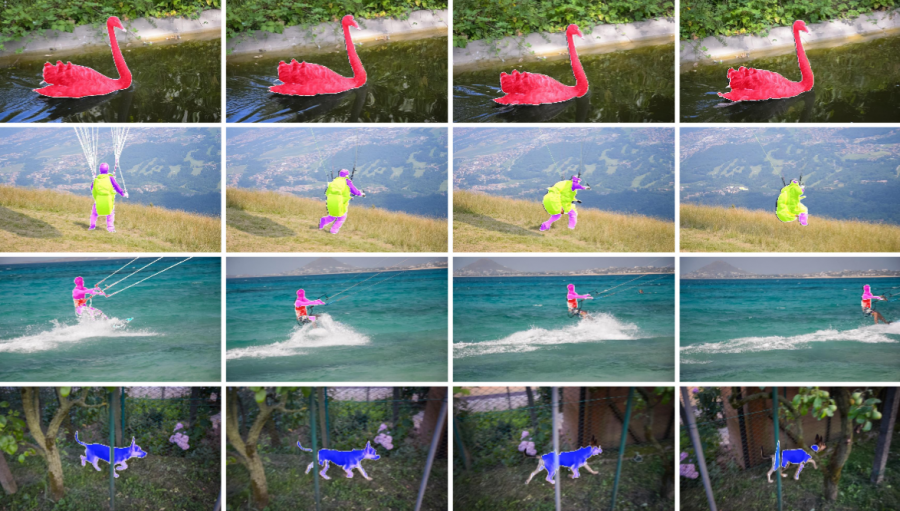
Researchers from the Department of Computer Science and Visual Geometry Group at the University of Oxford have proposed a new self-supervised learning method for video correspondence flow.
The task of video correspondence flow represents the task of matching correspondences between frames over a video. Researchers proposed a self-supervised approach which enables learning feature embeddings suitable for this task.
The proposed method is based on low-level color matching and researchers introduced a few novelties to tackle the problem in a more efficient manner.
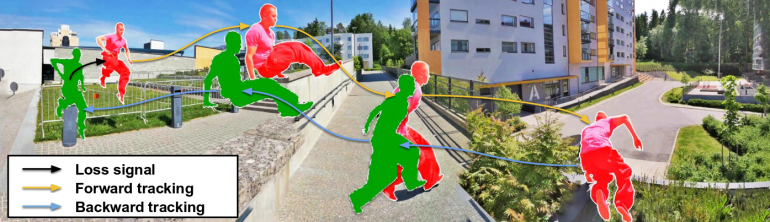
They introduced a so-called, information bottleneck while training a dense correspondence matching based on RGB coloring by removing image channels and adding jitter on the input images. By doing this, researchers wanted to enforce the elimination of trivial coloring solutions that would lead to potential bad frame matching. Another thing that researchers propose, is to use longer temporal windows and make the model recursive.
In the end, the model is performing a dense matching which is computed on rich feature embeddings of a very long temporal window and high resolution. The backbone network used within the method to extract features is ResNet-18.
The performance of the method was evaluated on several different tasks including video segmentation and keypoint tracking. Researchers showed the potential of the proposed approach which achieves state-of-the-art performance on DAVIS video segmentation. The method also outperforms other self-supervised methods on JHMDB keypoint tracking tasks.
The implementation of the novel video correspondence flow method was open-sourced and is available on Github. The paper is available on arxiv.