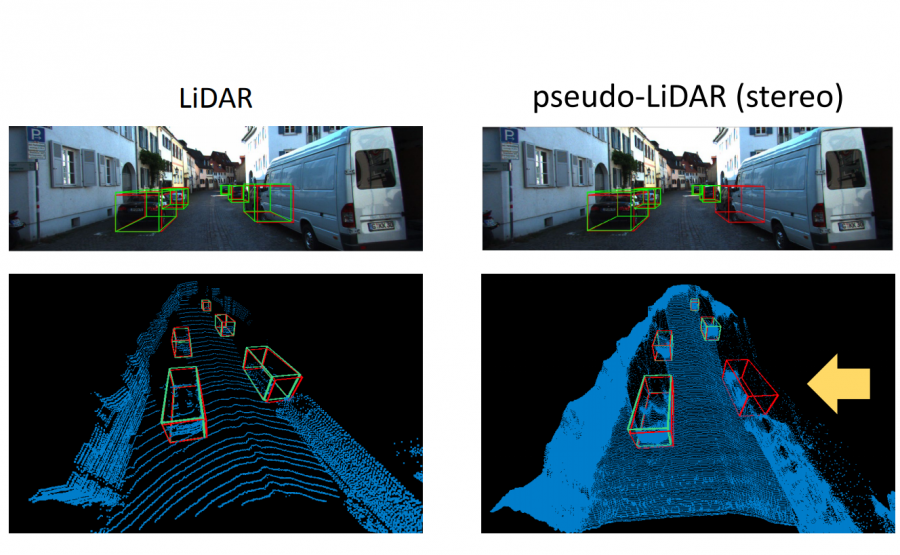
Researchers from Cornell University NY, led by Yan Wang have come up with an interesting idea on how to bridge the gap in accuracy between 2D and 3D object detection methods.
In their paper, titled “Pseudo-LiDAR from Visual Depth Estimation: Bridging the Gap in 3D Object Detection for Autonomous Driving”, they argue that it’s not the difference in data that makes 3D-based methods better but its representation. To demonstrate this, they propose a method for converting image-based depth maps into a pseudo-LIDAR representation (a representation that to some extent resembles the 3D point cloud coming from a LIDAR sensor).
In fact, researchers tried to mimic the LIDAR signal by using only camera data and depth estimation models. They propose a transformation of the image depth map to pseudo-LIDAR representation and use depth estimation and 3D detection model to overperform existing 3D detectors by a large margin.

The novelty that they introduce is the transformation from depth image to point cloud by projecting each pixel into a 3D coordinate. Instead of adding the depth as an additional channel in the image they try to derive a 3D location relative to the camera position.
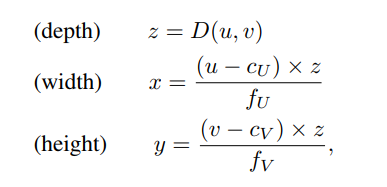
Researchers showed that the generated pseudo-LIDAR point cloud aligns well with the original LIDAR signal. The evaluations conducted on the popular KITTI benchmark show that this approach yields large improvements over existing state-of-the-art methods. The algorithm is currently the highest entry in the KITTI 3D object detection benchmark for stereo image-based methods.