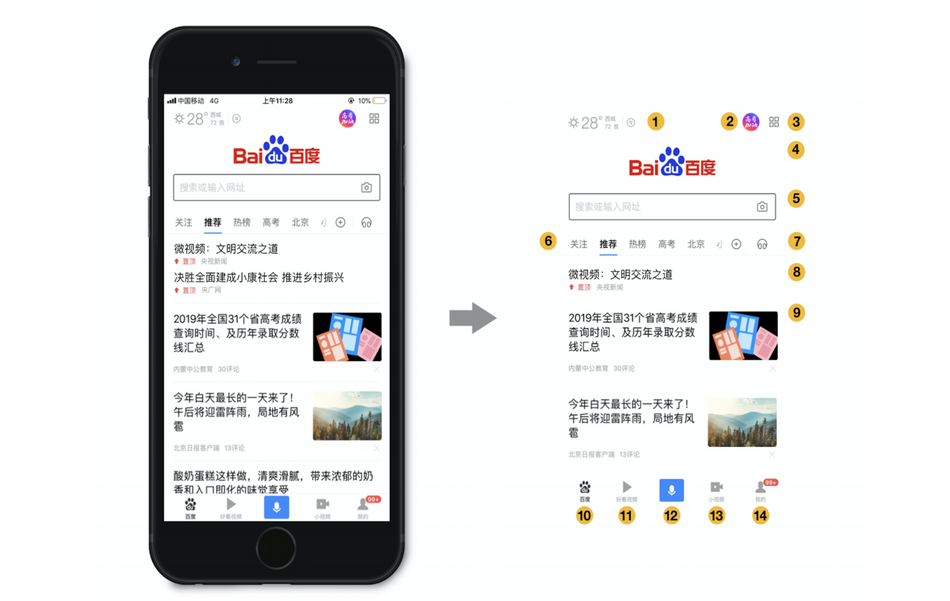
Researchers from Baidu in a collaboration with several universities and research institutes have developed an intelligent framework for the exploration of UI design solutions using collective machine learning.
The new framework, named FEELER can help designers and UI/UX experts to build novel UI modules and to quantitatively measure the preference score for their designed solutions. According to researchers, this is the first study that explores the application of machine learning methods for design exploration of user interface (UI) modules.
FEELER is a two-stage framework where in the first stage a proactive is trained with crowdsourcing labeled data. A group of participants is asked to rate a generated set of design solutions for a particular module. In this stage, researchers employ a Bayesian Active Learning approach to iteratively update the proactive model using the labeling from the crowdsourcing platform.
During the second stage, researchers build a so-called comparison-tuning model, based on the fact that users are better at comparing two design solutions than at scoring the preference for a single given solution. At this point, a pair of solutions generated by the proactive model is given to a participant in order to score the preference among the two given solutions.
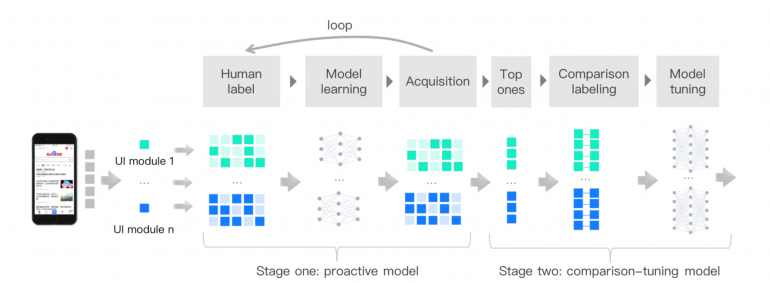
There were more than 500 participants taking part in the crowdsourcing data labeling to build FEELER over several labeling rounds. Researchers then conducted a few experiments using two real-world datasets and showcased the effectiveness of the new framework towards helping designers build better UI solutions.
More details about the framework and the experiments can be read in the paper published on arxiv.