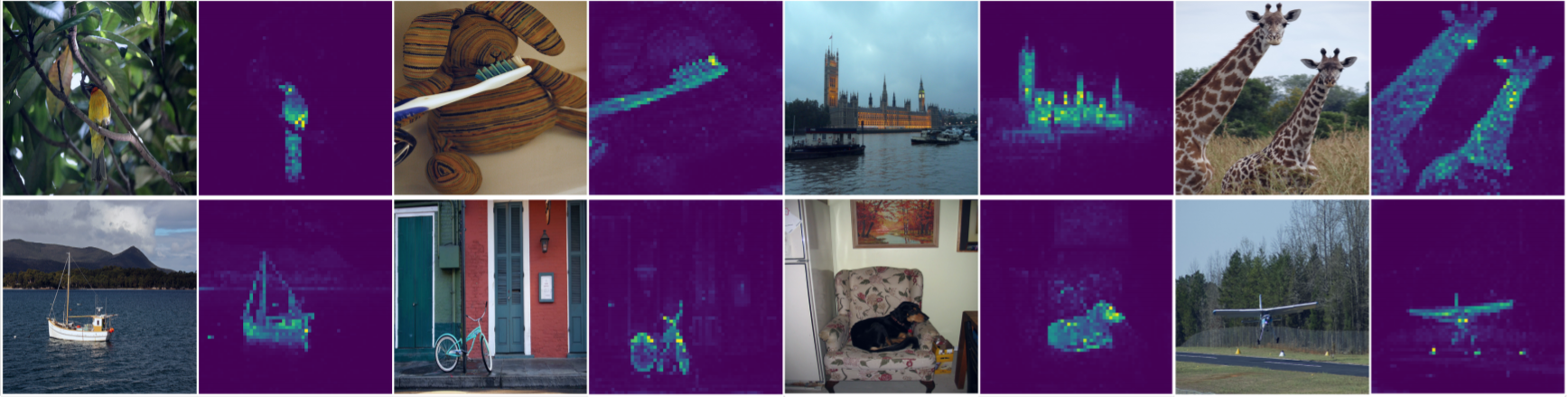
DINO is FAIR’s self-supervised tool for modern Visual Transformer models. The framework effectively handles highlighting of important content in images without labels. The open-source code is available.
Why is it needed
In general, visual segmentation accomplishes many tasks, from replacing the background in video chat to teaching robots to navigate the environment. It is one of the most challenging tasks in computer vision as it requires the AI to truly understand image content. Traditionally, the task is achieved through supervised learning and requires a large number of labeled examples. But DINO shows that high-fidelity segmentation can be accomplished with self-supervised learning and a suitable architecture. Using self-supervision with the Visual Transformer tool, DINO allows creating models with a much deeper understanding of images and videos.
How it works
The heart of DINO architecture is a learning paradigm called “knowledge distillation”. Simultaneously, two models are being involved, performing the roles of a student and a teacher. Both the student and the teacher receive the same image as input, slightly modified at random. The student model learns to fit the teacher model responses.
FAIR managed to adapt the knowledge distillation algorithm for self-supervision. The models are fed with patterns – random fragments of the image. The student receives both global and local patterns, and the teacher receives only global ones. This encourages the student to memorize the relationship between global and local patterns. Both models have the same structure. Stochastic gradient descent is used to fine-tune the student’s parameters. Key point: The teacher model is built based on the student model. The teacher’s internal parameters are periodically smoothly adjusted using an exponential moving average.
What’s the innovation
In addition to architectural innovations, it is noteworthy that DINO learns a lot about the visual world. By detecting image patterns, the model learns feature space with an explicit structure. If you use DINO to visualize categories from the popular ImageNet dataset, you can see that similar categories are next to each other. This suggests that the model can associate categories based on visual properties, just like people do. For example, it categorizes animal species in a structure that resembles biological taxonomy.