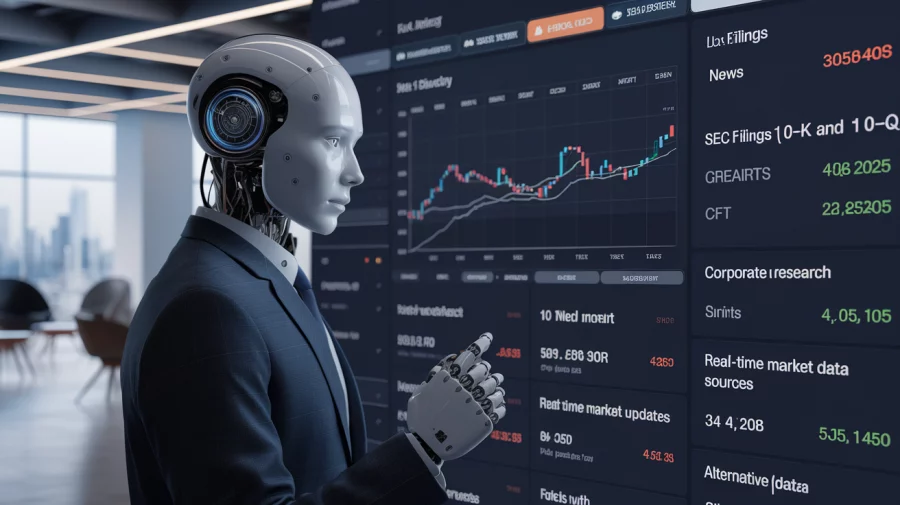
AI4Finance Foundation researchers have released FinRobot, the first AI agent framework specifically designed for equity research. Finrobot addresses key limitations of existing automated research tools by combining both quantitative and qualitative analyses through a multi-layer Chain of Thought (CoT) approach. This open source solution is available on Github.
The paper identifies that while Generative AI has attracted attention in financial analysis, existing AI solutions often fall short due to their narrow focus on technical factors and limited capacity for discretionary judgment. FinRobot aims to solve this by integrating comprehensive reasoning capabilities similar to human analysts.
The framework processes multiple data sources, including SEC filings (10-K and 10-Q reports), corporate releases, earnings calls, industry research, real-time market updates, and alternative data sources.
Technical architecture
The method implements a three-layer CoT framework that replicates the analytical depth and narrative skills of human analysts across data processing, concept generation, and report synthesis.
The Data-CoT Agent serves as the foundation, aggregating and processing diverse financial data from SEC filings, earnings calls, and alternative sources, while ensuring accurate integration of both quantitative metrics and qualitative insights.
The Concept-CoT Agent acts as the analytical core, emulating human analyst reasoning to contextualize data, perform competitive analysis, and derive actionable insights through financial models and sentiment evaluation.
Finally, the Thesis-CoT Agent operates at the synthesis level, organizing findings into professional equity research reports following sell-side research standards, complete with investment recommendations, financial projections, risk assessments, and valuation modeling. This layered approach enables FinRobot to maintain the depth and coherence characteristic of professional equity research while automating the entire analysis process.
FinRobot Performance Evaluation
The researchers conducted expert evaluations using a panel of investment banking analysts who rated the system on three dimensions:
- Accuracy: Averaged 9.5/10 (scores: 9-10);
- Logicality: Averaged 9.4/10 (scores: 9-10);
- Storytelling: Averaged 8.4/10 (scores: 7-10).
The evaluation included detailed criteria for each metric, with reviewers assessing aspects such as data precision, report structure, and narrative engagement. The system’s outputs were compared against reports from major brokerage firms to validate quality.
Known limitations
The paper acknowledges that the system primarily operates in English, requires verification of factual accuracy, may need human oversight for complex market scenarios.