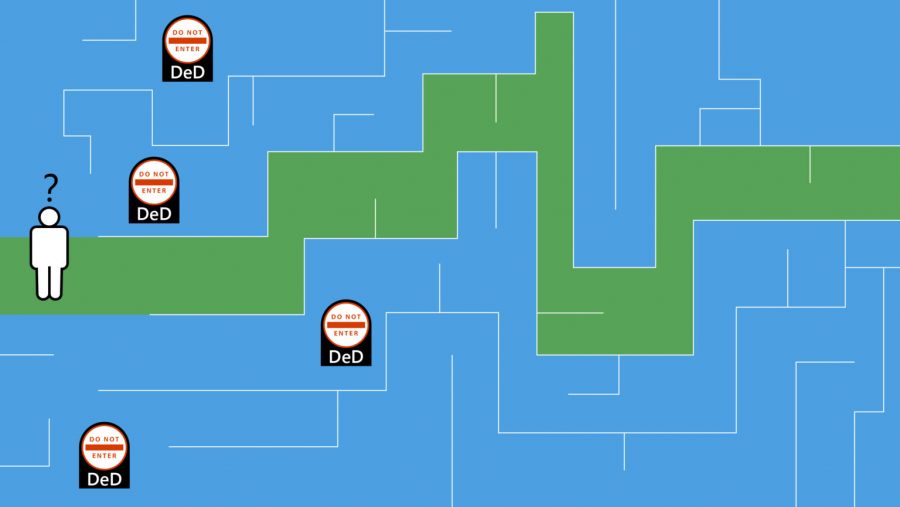
Microsoft has developed a reinforcement learning algorithm that offers the most effective treatment tactics for the patient’s current condition. The model is aimed at accelerating decision-making in healthcare in conditions of a limited amount of medical data.
The model was developed to recognize treatment protocols that can lead to negative results, and to warn doctors that the patient’s condition may worsen to a life-threatening level.
Healthcare is an area of sequential decision-making, and reinforcement learning is a formal paradigm for modeling and problem solving in such areas. In healthcare, doctors base their treatment decisions on a general understanding of the patient’s health; they observe how the patient responds to this treatment, and then the process repeats. Similarly, in reinforcement learning, an algorithm or agent interprets the state of its environment and performs an action that, in combination with the internal dynamics of the environment, causes it to move to a new state.
Microsoft’s algorithm identifies treatments that should be avoided to prevent a “medical impasse” — the point at which a patient is most likely to die regardless of future treatment. The peculiarity of this approach is the exponentially smaller amount of required data compared to other methods, which makes it significantly more reliable in situations with limited data. By highlighting high-risk treatments, this method can help doctors make reliable decisions in situations requiring an emergency response.