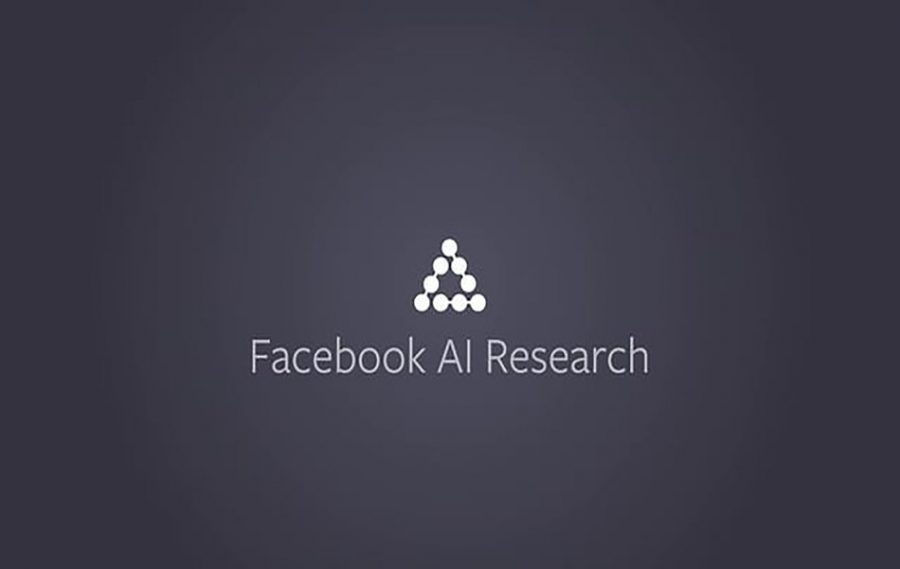
Facebook AI Research (FAIR) has announced the release of Libri-light – a new benchmark for automatic speech recognition (ASR) systems.
The new benchmark contains three types of datasets as part of the large training set. It contains 60K hours of unlabelled speech data mainly from audiobooks in English, together with a smaller limited supervision training set which consists of 10-hour, 1-hour and 10-min long parts of labeled data and an unaligned text extracted from the LibriSpeech LM dataset. The data within the large unlabeled dataset contains snippets mostly from literature, science, religion, and poetry.
In addition to the large datasets, Libri-light contains metrics, tasks, baseline models and also pre-trained models that were trained using the Libri-light datasets. In terms of tasks and metrics, the new benchmark was built to support research mainly within the three branches of unsupervised, semi-supervised and distantly supervised learning in automatic speech recognition systems. Researchers from FAIR implemented some of the commonly used metrics in these systems such as ABX-error (a discrimination metric used in an unsupervised setting), PER (phoneme error rate) and CER (character error rate), WER (word error rate) and others. They mention that all of the metrics can be used in nested fashion among the three training settings. The structure can be seen in the diagram below.
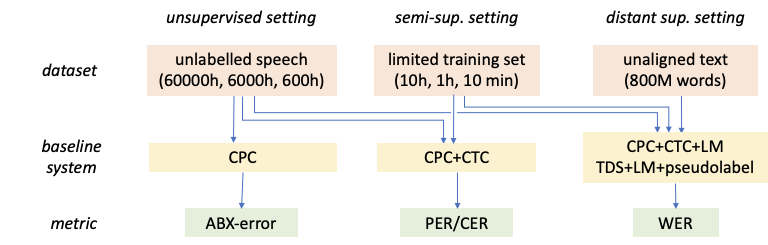
The code for training baseline systems was provided together with all the pre-trained and baseline models and can be found in Github. More in detail about the new ASR benchmark can be read in the paper or the official blog post from Facebook AI Research.