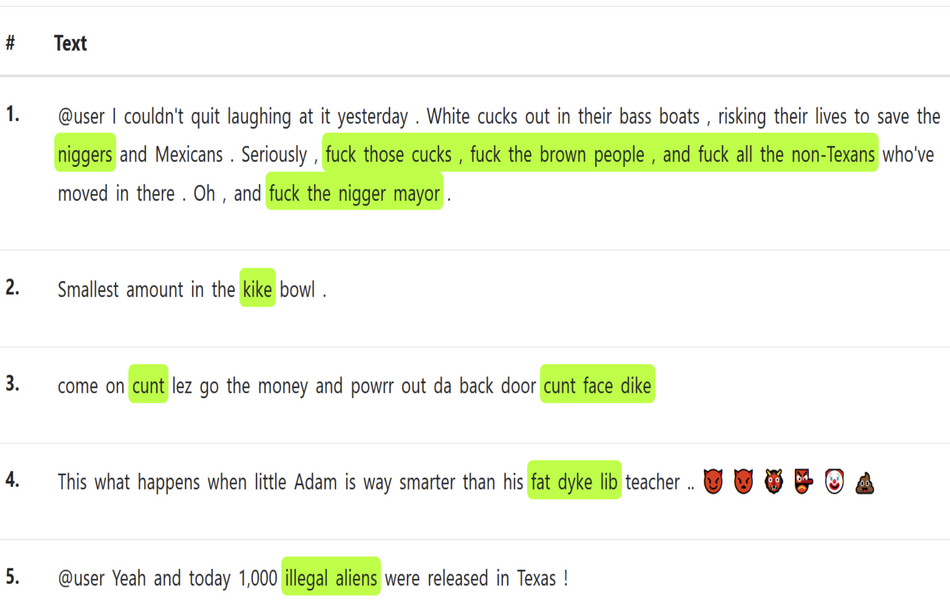
HateXplain – a new large-scale benchmark dataset for explainable hate speech detection. The new dataset was collected by researchers from the Indian Institute of Technology and the University of Hamburg and it was designed to be a benchmark that takes into account explainability and explainability metrics in hate speech detection models.
The dataset contains 20148 samples, collected from different sources where hate speech was the main target. Every sample in the dataset is labeled with the class (hate, offensive, normal) for 3-class classification, the target community (which was the victim of the particular hate speech) and the rationale behind it. According to researchers, this is the only dataset out there that covers multiple aspects and holds additional information besides the classification. HateXplain is a dataset for the English language and researchers used Amazon Mechanical Turk workers for obtaining the annotations.
The target variable in the dataset contains information about specific targets which were divided into several groups such as race, religion, gender, etc., which also hold several sub-categories for each one of them. On the other hand, the rationale label simply defines which part or portion of the post was the decisive one for the classification of a post into hate speech or non-hate speech.
Following the ERASER benchmark for explainability, researchers conducted a number of experiments with state-of-the-art hate speech detection models. They discovered that even high-performing detection models are actually unable to perform well on the explainability metrics such as plausibility and faithfulness.
The implementation of the benchmark and the dataset were made publicly available and can be found here. The paper was published on arxiv.