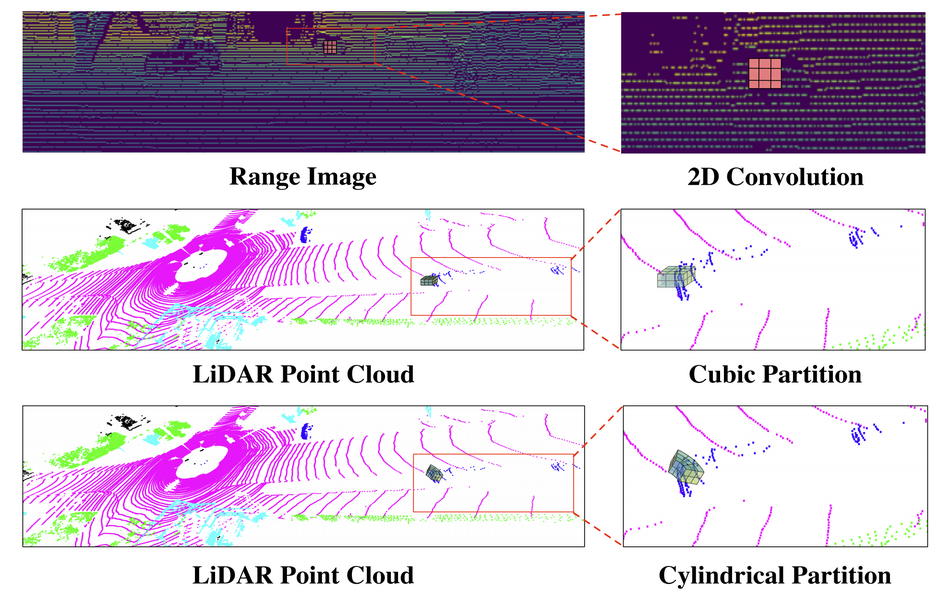
A group of researchers from several Chinese universities has developed a novel state-of-the-art method for LIDAR semantic segmentation using asymmetrical 3D convolutional networks.
In their paper, “Cylindrical and Asymmetrical 3D Convolution Networks for LiDAR Segmentation” researchers describe the limitations of existing lidar segmentation methods which use both 2D and 3D convolutional neural networks. They also propose a new method that overcomes those limitations, namely the point cloud sparsity and the variable and non-uniform point density. They mention that in contrast to indoor scenes, outdoor lidar scans have very specific point distribution and they attribute most of the limited performance of existing methods to this particular characteristic.
Researchers proposed a new 3D representation of the LIDAR point cloud data along with a new segmentation framework and a novel neural network architecture. Instead of the traditional voxelization, researchers propose to use so-called cylindrical partitions which allow for a more uniform point distribution across partition cells. They defined a specific architecture that leverages that representation in order to extract high-level meaningful features that capture 3D space information.
The proposed segmentation framework consists of two branches: voxel-based learning, which takes advantage of the cylindrical representation, and a high-level detail branch which extracts point-wise features from the input point cloud. The first branch employs the proposed “Asymmetrical Residual Block” in an encoder-decoder structure while the other branch uses an MLP to extract features for each point. The architecture is depicted in the image below.
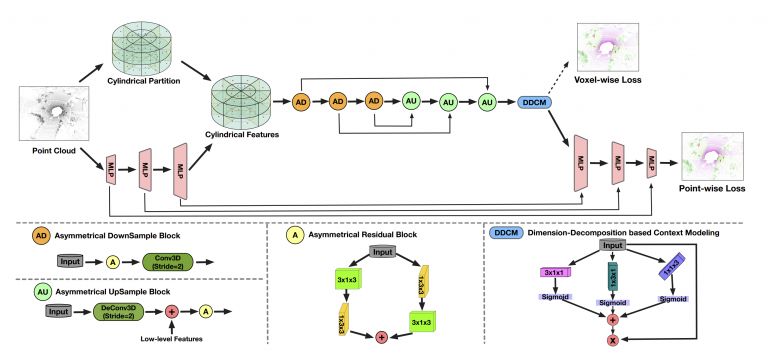
Researchers conducted extensive experiments on two large-scale datasets: nuScenes and SemanticKITTI. Quantitative results showed that the method outperforms all existing methods on the SemanticKITTI dataset achieving an mIoU score of 63.5.
More details about the method, the conducted experiments, and the results can be found in the paper. The implementation was open-sourced and it is available on Github.