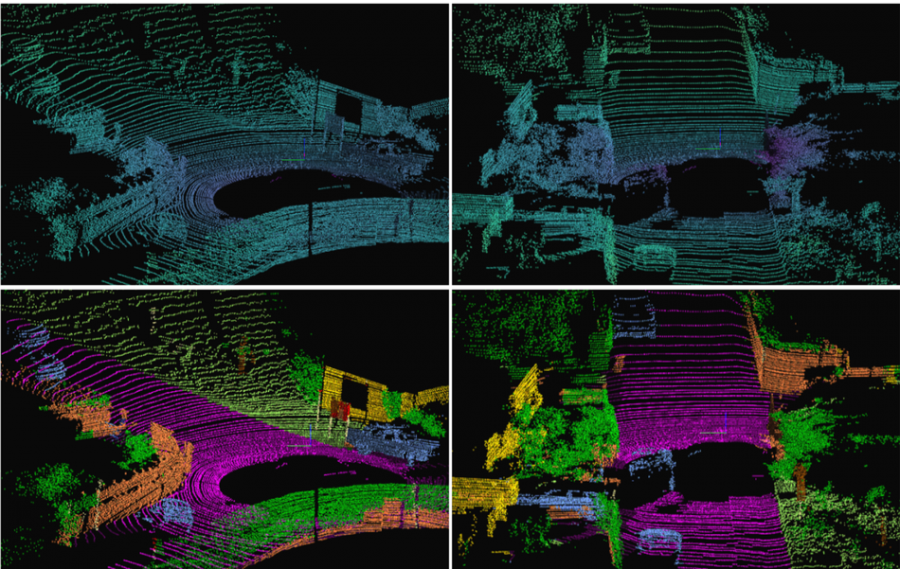
A group of researchers from the University of Zaragoza, has proposed a novel method for LIDAR semantic segmentation that achieves state-of-the-art performance on popular public segmentation benchmakrs while being more computationally efficient than other existing methods.
The key idea that researchers explore is the learning of a 2D representation from LIDAR’s point cloud in a fast and efficient manner and then using this representation to perform the segmentation using 2D convolutional networks. In the paper, researchers argue that fast and efficient algorithms for semantic segmentation of 3D point clouds are necessary for tackling many real-world problems in domains such as robotic navigation and autonomous driving.
The new model, named 3D-MiniNet consists of two main parts: a learning module, which learns to extract a 2D tensor from the input point cloud and a segmentation module, which is basically an efficient fully convolutional neural network that performs segmentation in 2D space. The semantic labels given by this 2D segmentation model are then re-projected back to 3D and post-processed using KNN in order to obtain the final 3D point cloud. The whole model was trained on KITTI datasets and using simple data augmentation techniques such as random rotations and shifting of the point cloud.
The evaluation of the method was done using the two well-known benchmarks: SemanticKITTI benchmark and the KITTI benchmark. The results showed that 3D MiniNet is superior to existing methods both in terms of semantic segmentation performance, and at the same time being two times faster and having 12 times fewer parameters. Researchers also performed an ablation study in order to investigate the contribution of each of the components in the final result.
More about 3D MiniNet can be read in the pre-print paper, published on arxiv.