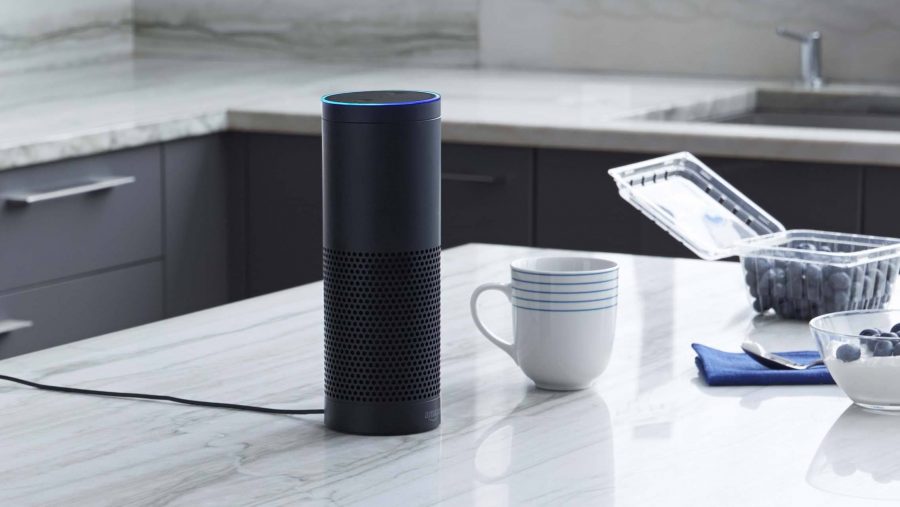
Amazon’s voice assistant speaker Echo, backed by its controller Alexa, now in its second generation and with several derivative versions available, continues to expand its music, smart-home, and digital-assistant capabilities. The voice control system that lets you speak your wishes to your devices is now capable of doing many things.
Backed by Artificial Intelligence and Natural Language Processing (NLP) Alexa can help in many different ways. Amazon’s technology behind Echo and Alexa is mature enough to allow the system to perform these tasks.
Recently, Rohit Prasad, vice president and head scientist of Alexa AI at Amazon has revealed some details from behind the scenes and the technology that powers Alexa. In a press release, he explained most of the features of Alexa backed by Machine Learning and large-scale AWS cloud computing power. He discussed five areas of research relevant to Alexa’s development and progress: competence, context awareness, knowledge, natural interaction, and self-learning.
One of the recent advances and features of Alexa is intelligent parsing coordination. Or in simple terms: “how Alexa knows “Peanut Butter” is one shopping-list item, not two.”
Researchers from Amazon have published a paper recently describing a new deep neural network-based parser.
“We augment an SLU system with a domain-agnostic parser that can identify syntactic elements that establish relationships between different parts of an utterance, or “coordination structures.” In tests comparing our system to an off-the-shelf broad syntactic parser, we show a 26% increase in accuracy.” says Alexa’s head scientist.
The new parser is a deep neural network that is trained on varied coordination structures in spoken-language data. The training examples are labeled according to the BIO scheme, which indicates groups of words — or “chunks” — that should be treated as ensembles. This allows solving the problem of considering “Peanut Butter” as a single item, for example.
The deep neural network used is a bi-directional LSTM able to learn from the word and character-level embeddings. However, the best results obtained came from a model that used character embeddings, pretrained FastText embeddings, and a CRF layer. The paper is published on arXiv, and more information can be read at Amazon’s blog post.