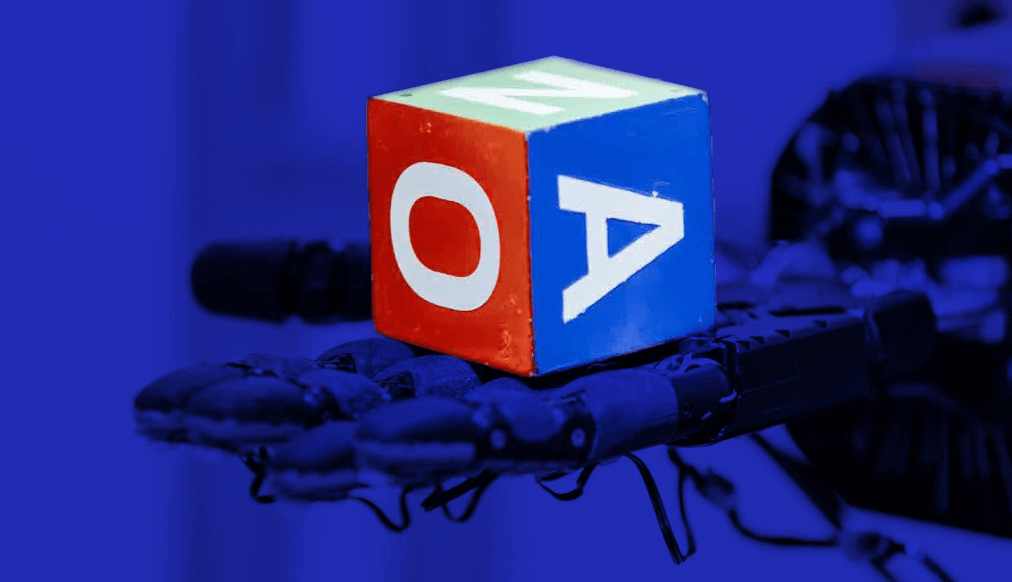
It has been observed that when using large batch sizes there is a persistent degradation in generalization performance – known as the “generalization gap” phenomenon. There has been a huge discussion among researchers, engineers and other people in the machine learning community on how to treat the batch size parameter and if smaller or larger batches work better or worse in specific cases.
Researchers from OpenAI (part of the OpenAI Dota Team) have published a paper with important findings on how AI training scales and large batch sizes. In fact, they discovered that the gradient noise scale, a simple statistical metric, predicts the parallelizability of neural network training on a wide range of tasks.
They found out that there is a positive correlation between Gradient Noise Scale and batch size. When the gradient noise scale, a simple statistic that quantifies the signal-to-noise ratio of the network gradients is small, learning from a lot of data in parallel quickly becomes redundant. However, when the gradient noise is large, we can still learn a lot from large(or huge) batches of data.
This allowed the researchers to approximately predict the maximum useful batch size. They verified their claim (or prediction) for a wide range of tasks like image recognition, language modeling, Atari games, and Dota.
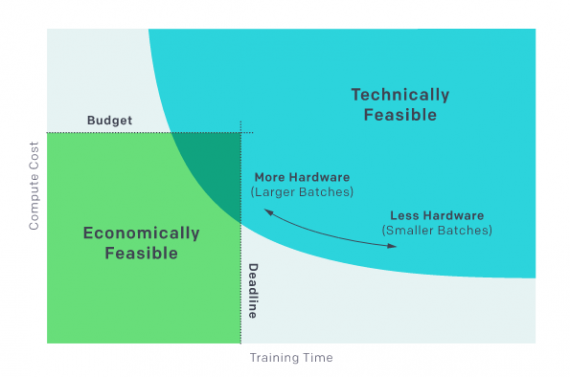
In the paper, they further explore the problem of scaling AI, both from technology and economy perspective. Also, they investigate the Gradient Noise Scale metric and provide useful insights in the form of observed patterns. The researchers believe that this might hold the key to answering the difficult questions about neural network training. As they say, this is just one proof that deep neural network training can be systematically explained and it should not be considered a mysterious art. More about their discovery can be read on OpenAI’s blog post. The paper is published at arxiv.