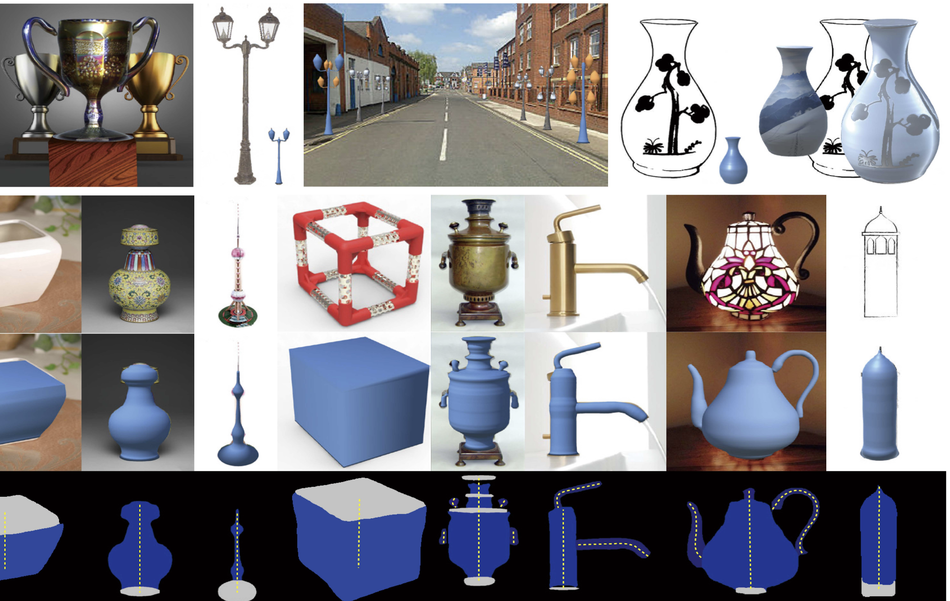
A group of researchers from the Shangai Tech University, University of Leeds, and Zhegjiang University have developed a deep neural network for recovering 3D editable objects from a single image.
Their proposed method, named AutoSweep builds upon the assumption that most human-made objects consist of semantic parts which can be described by using generalized primitives. In order to recover these parts and fully editable 3D objects, researchers designed a novel segmentation network which outputs instance masks labeled as semantic parts. These parts are described as part-level masks that are labeled as either profiles or bodies. After the labeling of the separate parts, the outputs are passed to the next stage of the pipeline which extracts trajectory axes in order to identify profile-body relations and recover the final 3D parts of the object. The whole pipeline is shown in the diagram below.

In order to train and evaluate the model, researchers used both synthetic and real data. They curated a dataset of human-made primitive-shaped objects from several publicly available datasets among which the widely used ImageNet. The results of the conducted experiments showed that AutoSweep is capable of reconstructing 3D objects which are made of primitives such as generalized cuboids and generalized cylinders. Comparisons with existing methods show that the proposed framework for reconstructing 3D objects is superior in both instance segmentation as well as 3D reconstruction.
The implementation of AutoSweep was open-sourced and it is available on Github. The dataset was also published and can be found here.