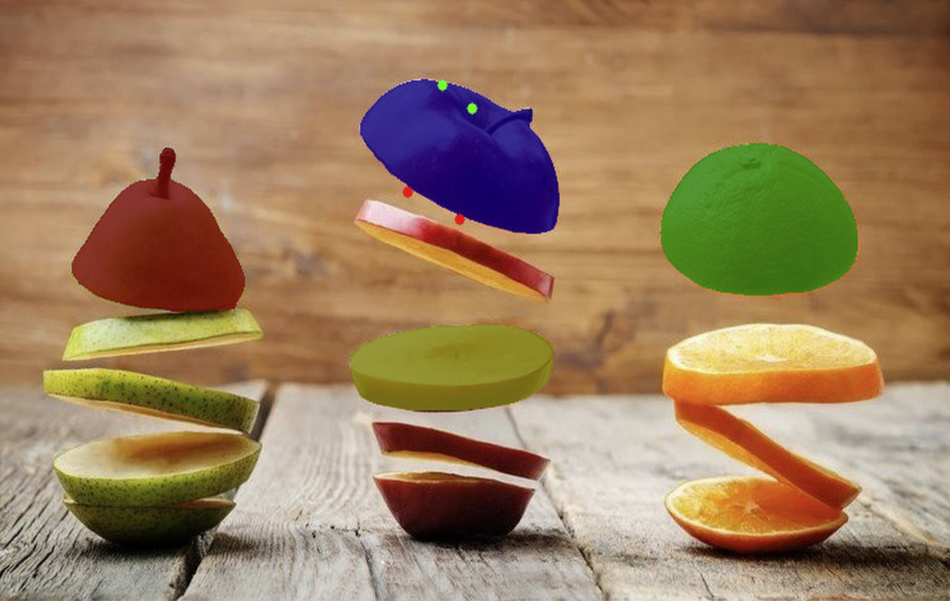
In a novel paper published on this year’s CVPR2020, researchers from the Samsung AI Center in Moscow led by Konstantin Sofiiuk, describe their new method for interactive segmentation named f-BRS.
The method builds upon previous advancements in the field of interactive segmentation, or more precisely the recently proposed “backpropagating refinement scheme” (BRS). Researchers observe that the vanilla version of this scheme is computationally inefficient since it requires multiple forward and backward passes through the segmentation network. To overcome this issue, the proposed f-BRS or feature backpropagating refinement scheme needs forward and backward passes only for a small region of the network.
Researchers experiment with their approach using the popular and powerful DeepLabV3+ segmentation model. In the architecture of DeepLab, they introduce the f-BRS scheme at three different places: after the ResNet backbone, after ASPP, and after the separable convolutions.
The proposed method has established itself as the new state-of-the-art method for interactive segmentation improving the cost per click (or time per click) by an order of magnitude over previous methods that employ BRS. The evaluations were performed using several well-known benchmark datasets: SBD, GrabCut, Berkeley, DAVIS.
The implementation of the method was open-sourced under the Mozilla MPL-2.0 license and can be found on Github. More in detail about the proposed method can be read in the paper.