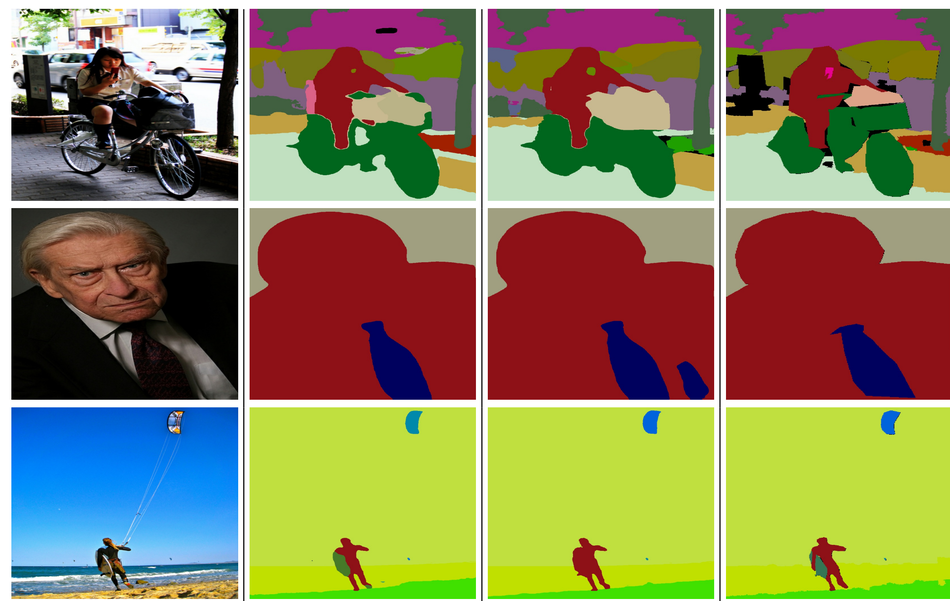
In their recent paper, researchers from Google AI have proposed a new state-of-the-art model for panoptic and semantic segmentation.
The new model comes as an improvement over the series of models labeled as “DeepLab” and proposed by Google researchers in the past few years. Researchers explored the so-called fully attentional networks in which self-attention layers are stacked and they suggest an improved architecture where they replace the more (computationally) expensive 2D self-attention with 1D local self-attention.
They introduce a novel layer that they call “axial-attention layer” and which consists of basically 1D position-sensitive self-attention. Consequently, the proposed network architecture employs so-called axial-attention blocks, which are simply blocks of two axial-attention layers (along width and height of the feature maps) preceded and followed by a 1×1 convolutional layers. The diagram below shows the structure of this block.
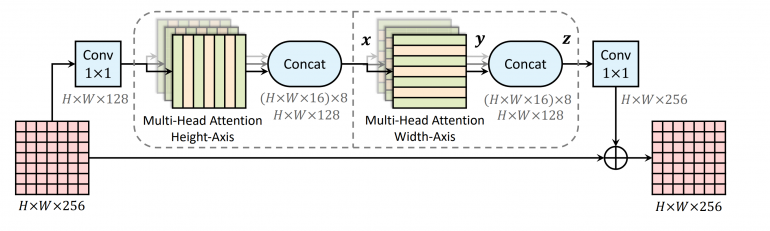
The new model was evaluated using four large-scale image datasets: Imagenet, COCO, Vistas, and Cityscapes. Researchers modified the popular ResNet model with included axial-attention blocks and they trained the model using the ImageNet dataset. Using this as a backbone, they experimented with the DeepLab architecture for the task of semantic and panoptic segmentation.
Results showed that the model outperforms all self-attention models on the ImageNet dataset, while on the tasks of panoptic segmentation it sets the new state-of-the-art score on the three datasets: COCO, Mapilary Vistas, and Cityscapes.
More about the new Axial-DeepLab model can be read in the blog post or in the paper published on Arxiv.