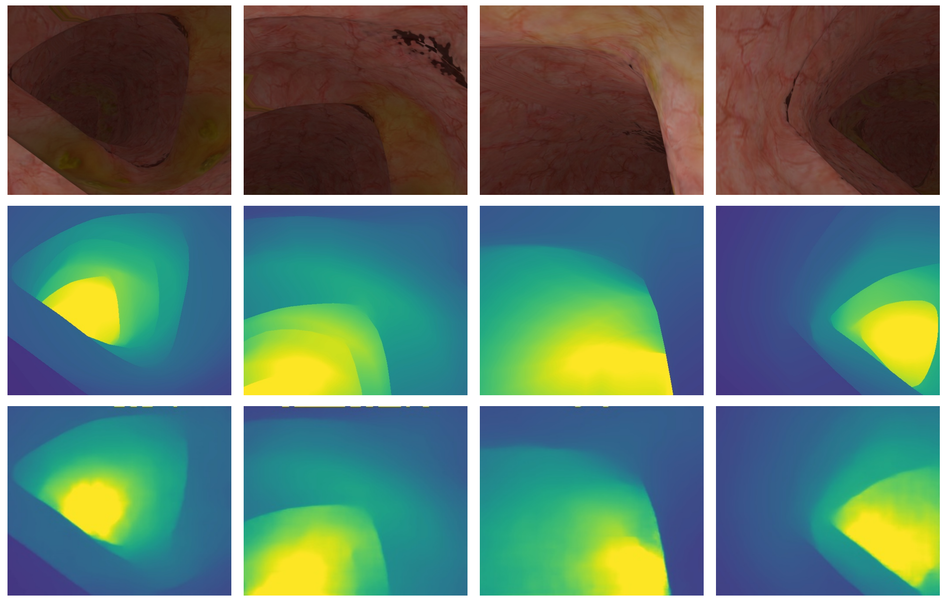
In another research project from Google AI, researchers have developed a machine learning method that helps doctors increase coverage in colonoscopy screenings.
Colonoscopy as a procedure for detecting polyps and tumors within the intestines is not perfect and very often doctors or endoscopists miss smaller or larger parts during the screening. In their novel paper, researchers try to tackle exactly this problem by employing machine learning techniques. They have proposed and developed an algorithm called C2D2 which helps to detect deficient coverage in the screening procedure.
The method basically creates a 3D local model of the colon by using the sequential image stream from the endoscope together with a depth prediction model to identify areas that might have potentially remained outside of the view. It works in real-time, so the method basically assists the endoscopist during the procedure by reconstructing a 3D model as the procedure goes.
The proposed network architecture consists of two branches: depth estimation branch and pose estimation branch. Two incoming images are fed into the network: one through the depth branch and the two combined into the pose estimation branch. The whole network is then optimized by employing a view synthesis loss function. A scheme of the architecture is given in the diagram below.
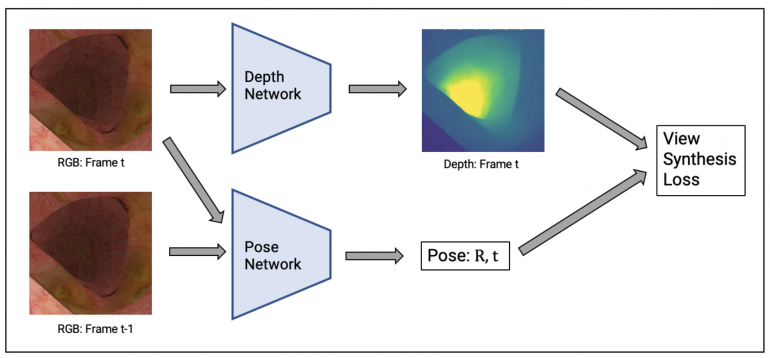
Researchers collected training data by using a synthetic data generator where they generated 3D models of the colon and asked physicians to label the videos, so that they can compare with the ground truth. Compared with the ground truth on synthetic videos, C2D2 algorithm outperforms physicians by a large margin. On real videos, it has 93% agreement with physicians.
More in detail about the method, data collection and results can be read in Google’s official blog post or in the paper.