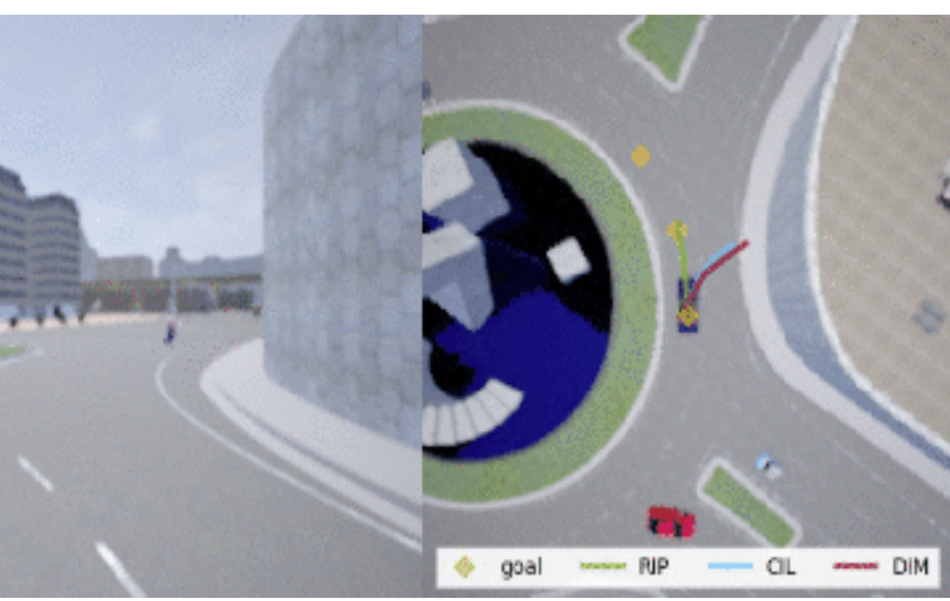
A group of researchers from the University of Oxford has published a paper in which they try to answer the question: Can Autonomous Vehicles identify and adapt to distribution shifts?
Autonomous vehicles being developed recently, are heavily using machine learning and deep learning models to model the surroundings and afterward to make informed decisions about what actions to take. However, these models are often trained with large but still limited amounts of data, and there is no guarantee that these datasets contain most of (or all) possible traffic situations, making it uncertain if autonomous vehicles can operate safely in some new, previously unseen scenario.
This, so-called distribution shifts were analyzed by the researchers from the perspective of autonomous vehicles. Researchers proposed a solution for the OOD (out of distribution) type of uncertainty within the framework of imitation learning.
Their algorithm is a type of deep imitative model, which follows the evaluate and plan paradigm. The robust algorithm differs from previous approaches in two main things: it maintains a belief of the model parameters which defines a distribution over future trajectories and it captures epistemic (model) uncertainty at the evaluation step before planning.
In order to improve the newly proposed probabilistic model, researchers propose an additional adaptation that uses access to an “expert” when out-of-distribution scenes are encountered. They name this method as AdaRIP.
To showcase the potential of the proposed method, researchers implemented their method and evaluated it using two datasets: nuScenes and CARNOVEL (synthetic data from Carla simulator). The final conclusions were that the method performs well and can detect OOD samples/scenes and update it’s internal model accordingly. However, according to researchers, there are still many open questions regarding time criticality, adaptation, etc.
More details about the proposed method can be read in the blog post.