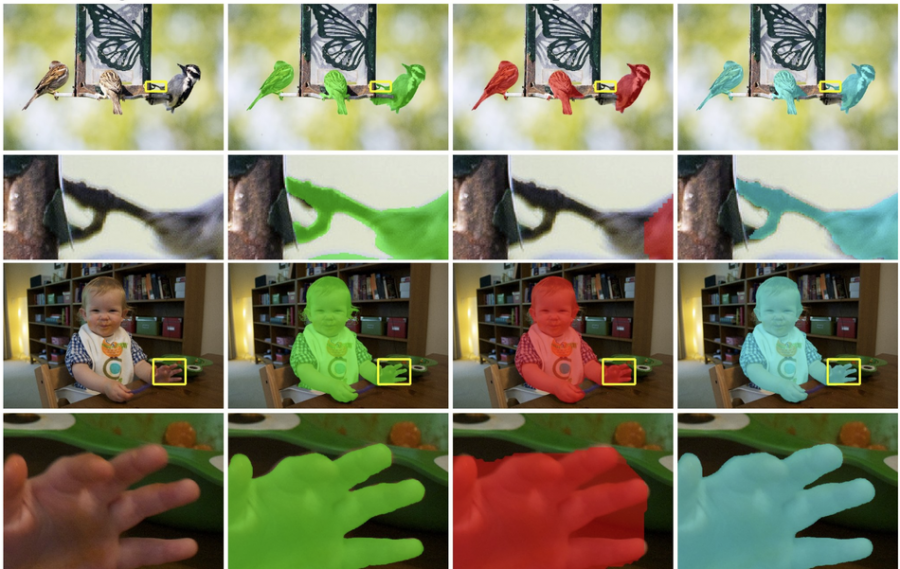
A group of researchers from the Hong Kong University of Science and Technology and Tencent have proposed a new segmentation model that provides high-resolution semantic segmentation without the need of any finetuning.
In their recent paper, researchers describe a new method, namely a new segmentation model with global and local refinement modules that performs well even on images larger than 4K. They argue that existing methods for semantic segmentation perform poorly on high-resolution images, mainly because they were trained on images with lower (and fixed) resolution range. To this extent, the upsampling method variants do not solve the problem of imprecise segmentation and therefore methods fail to capture high-resolution details and consequently object boundaries.
In order to overcome this problem, researchers designed a new model called CascadePSP. The main building block of their model is the novel refinement module. This module takes an input image along with multiple “coarse” and imprecise segmentation masks produced at different scales in order to generate a refined segmentation. The refinement module uses a ResNet encoder part and pyramid pooling, before upsampling the output to several different scales and providing a refined segmentation. Researchers did an ablation study to analyze the advantages and shortcomings of the introduced module and concluded that by using a multi-level cascade, the module delegates different refinement tasks to different scales.
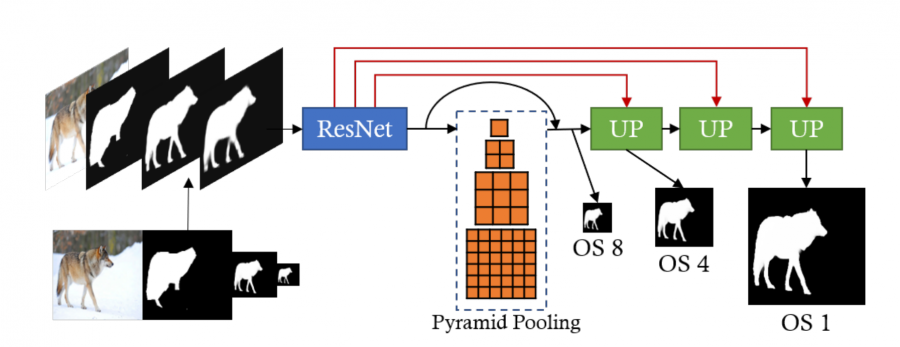
The final method uses both global and local refinement steps. During the global step, the whole image is fed into the module after downscaling it to the extent of fitting in GPU memory. Then, during the local steps image crops from a higher resolution image are passed through the refinement module.
Experiments show that the proposed method is able to perform precise semantic segmentation of high-resolution images, regardless of the size of the image. Researchers report consistent improvement over the existing state-of-the-art methods across several datasets.
The implementation of the method was open-sourced and it is available on Github. More details about the method can be read in the paper published on arxiv.