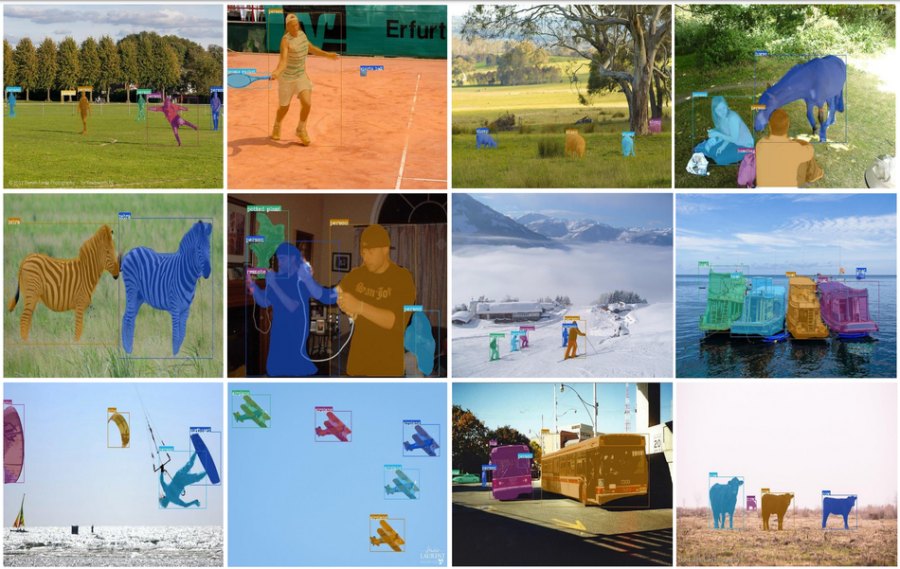
Researchers from the Electronics and Telecommunications Research Insitute of Korea have proposed a new state-of-the-art method for instance segmentation.
The novel method called CenterMask is based on a fully-convolutional one-stage object detector (FCOS) which is modified to include a new spatial attention mask – SAG-Mask. In the proposed architecture there exists a special SAG-Mask branch that outputs the segmentation mask for each bounding box together with a spatial attention map. According to researchers, this allows to decouple detection and segmentation as well as to filter out noise by focusing on more important pixels, given the attention map.
Together with CenterMask, researchers propose a small version of the model called CenterMask-Lite. This version was designed to run instance segmentation in real-time (more than 30fps). The original CenterMask was downsized in several dimensions. The backbone network, the head and mask head were downsized and the backbone was changed to VoVNetV2.
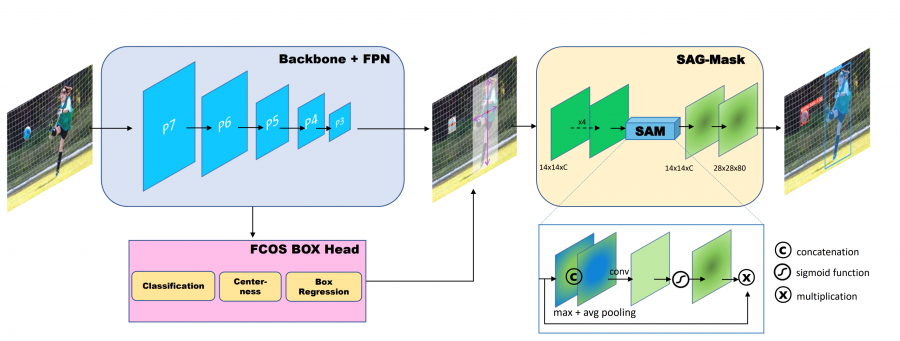
Researchers conducted experiments using ResNet-101 as a backbone network and evaluated the method on the popular COCO benchmark dataset. The evaluations showed that CenterMask outperforms all state-of-the-art models in instance segmentation. Also, CenterMask-Lite outperforms all existing methods on the COCO benchmark for over 35fps on Titan GPU.
The implementation of the novel method was open-sourced together with pre-trained models and is available on Github.