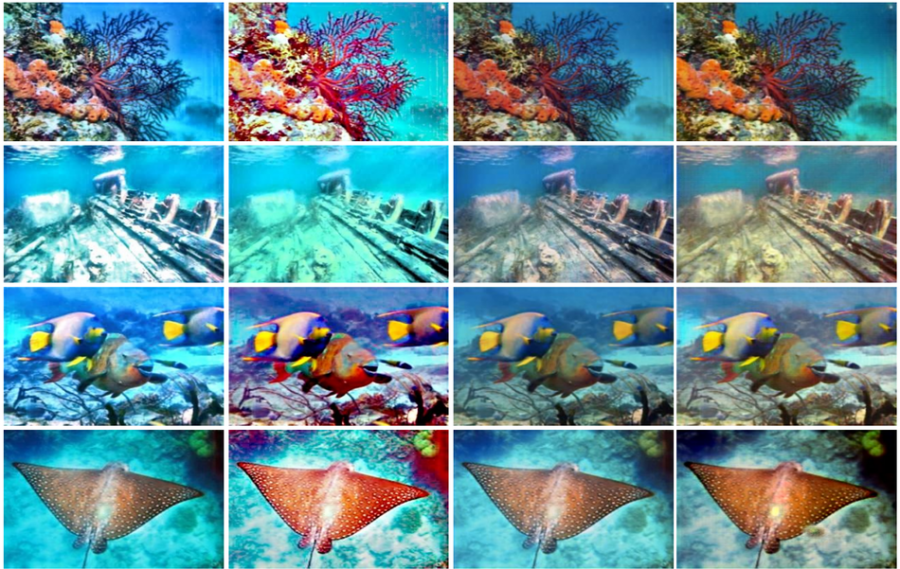
A group of researchers from the Minessota Robotics Institute has proposed a novel method that uses deep learning for enhancement and super-resolution of underwater images.
In their paper, named “Simultaneous Enhancement and Super-Resolution of Underwater
Imagery for Improved Visual Perception”, the group explores the problem of super-resolution for underwater robots’ vision and describes a generative model that can restore perceptual image quality of underwater images.
The problem of underwater image recovery and enhancement is a well-known challenge for many years and researchers have tackled this problem using traditional techniques as well as deep neural networks. In this work, researchers developed a method that simultaneously tackles both image enhancement and super-resolution in a so-called SESR framework. The method was called DeepSESR and it’s a generative model that learns how to restore perceptual qualities with up to 4 X spatial resolution.
Researchers designed a specific loss function that incorporates things such as underwater color degradation, lack of sharpness, etc. Additionally, researchers force the network to learn a saliency map by providing a supervisory signal on salient foreground regions in the training images. This, in turn, allows for image enhancement by enforcing global contrast enhancement. The architecture of the method contains a feature extraction network and three separate branches that learn: saliency maps, image enhancement and finally image super-resolution.
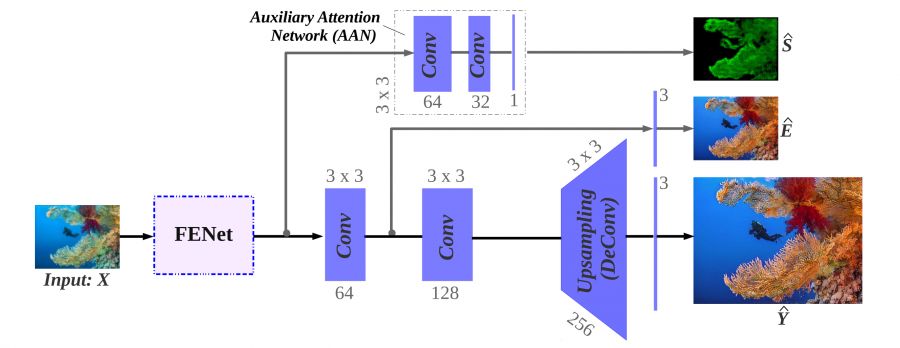
The model was trained in an end-to-end manner and evaluations showed that the method outperforms current state-of-the-art methods in underwater image enhancement and image super-resolution.
A pre-trained model along with some dataset information will be available on Github. More about the method can be read in the paper published on arxiv.