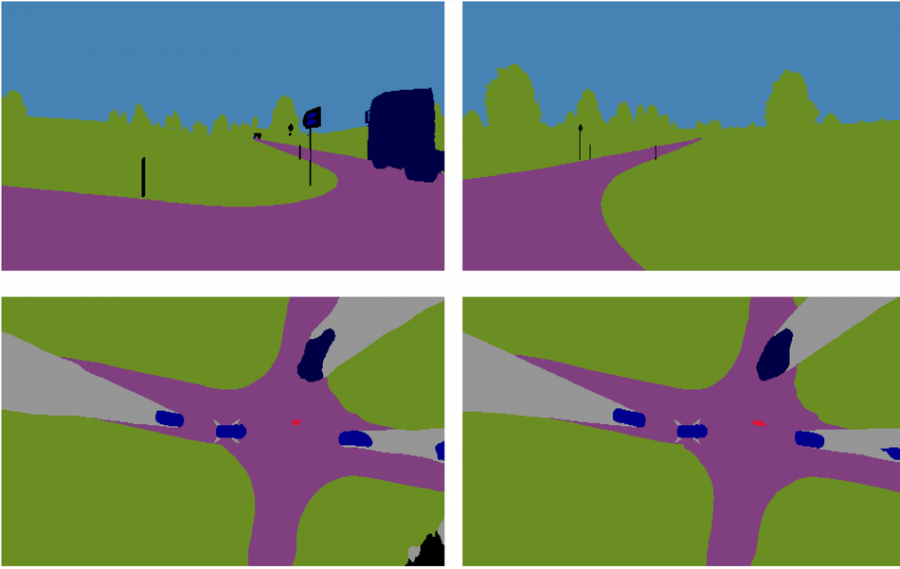
A group of researchers from the RWTH Aachen University has proposed a deep learning based method for transforming on-road images to semantically segmented bird-eye view (BEV) image.
Semantic segmentation has gained a lot of attention in the past years as it provides meaningful information for scene description and understanding. This is especially the case in Autonomous Driving where knowledge of the environment is essential for accomplishing the task of autonomous operation. In this context, getting depth information or transforming monocular camera images to bird-eye view from the scene has enormous potential, but also represents a challenging task.
To solve this problem researchers from Aachen University, propose a deep learning framework in which they leverage synthetic data to train a deep neural network model to predict correct BEV segmentation masks from mono camera images. In their proposed approach, several camera images from on-vehicle cameras are segmented and fed into the neural network which outputs a correct BEV segmentation mask. The model, called uNetXST consists of several encoders or encoder paths for the different input images and spatial transformer modules, whose outputs are concatenated to generate the final segmentation mask.

The network was trained using a synthetic dataset created using the virtual simulation VTD (Virtual Test Drive) and researchers managed to collect around 33 000 training samples and 3700 validation samples.
The evaluations of the method showed that it is capable of transforming the input images to precise BEV segmentation masks and that it performs superior to IPM.
Details about the method along with the conducted experiments can be found in the paper published on arxiv.