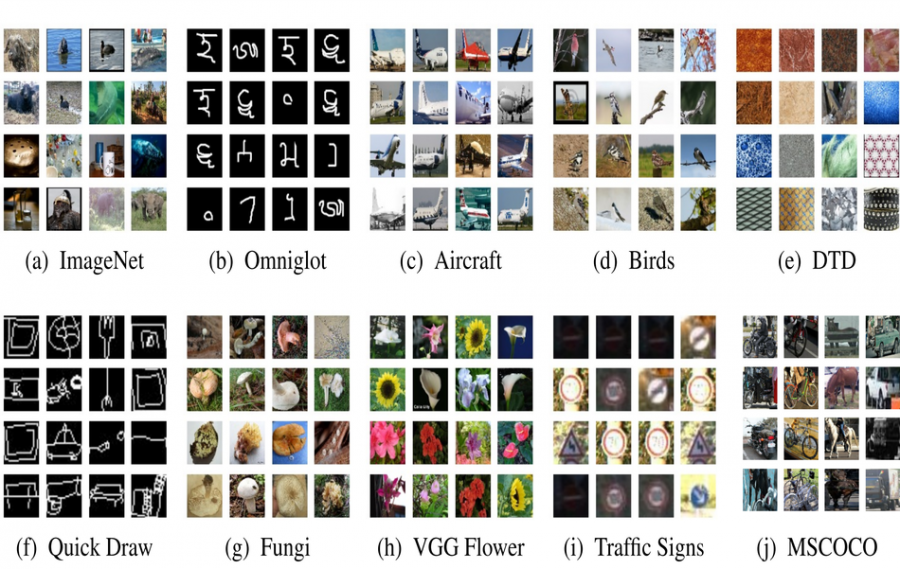
Researchers from Google AI have developed and released a new dataset for few-shot learning called Meta Dataset. The novel dataset is in fact attributed as a “dataset of datasets” and it integrates publicly available datasets of natural images, in a benchmark framework for measuring the performance of models in few-shot learning.
The goal of Meta Dataset was to improve upon existing benchmarks for few-shot learning and provide a platform for more extensive experimentation and a more realistic evaluation of models trained in a few-shot learning setting. In this context, researchers created Meta Dataset to be a large-scale dataset, by combining a lot of already big datasets among which ImageNet, Fungi, and others. The proposed benchmark on top of Meta Dataset, features realistic class imbalance and it significantly varies the number of classes in each of the tasks.
According to the researchers, they combined data from publicly available, easy-to-access datasets that can together contribute to the creation of a diverse dataset with a wide variety of visual concepts inside. The following 10 datasets were included in Meta Dataset: LSVRC-2012 (ImageNet), Omniglot , Aircraft , CUB-200-2011 (Birds), Describable Textures ,Quick Draw , Fungi , VGG Flower, Traffic Signs and MSCOCO. Out of these datasets, TrafficSigns and MSCOCO were left out of the training set completely and were reserved as testing datasets.
Along with the creation of the dataset, researchers performed an experimental evaluation of popular models and have defined a new set of baselines. Additionally, they did an analysis of how different models behave when trained with more data, heterogeneous data, and meta-training, and they released a novel meta-learner that according to them performs well on the new dataset.
More details about the data collection, the dataset, and the new benchmark and analysis can be read in the published paper.