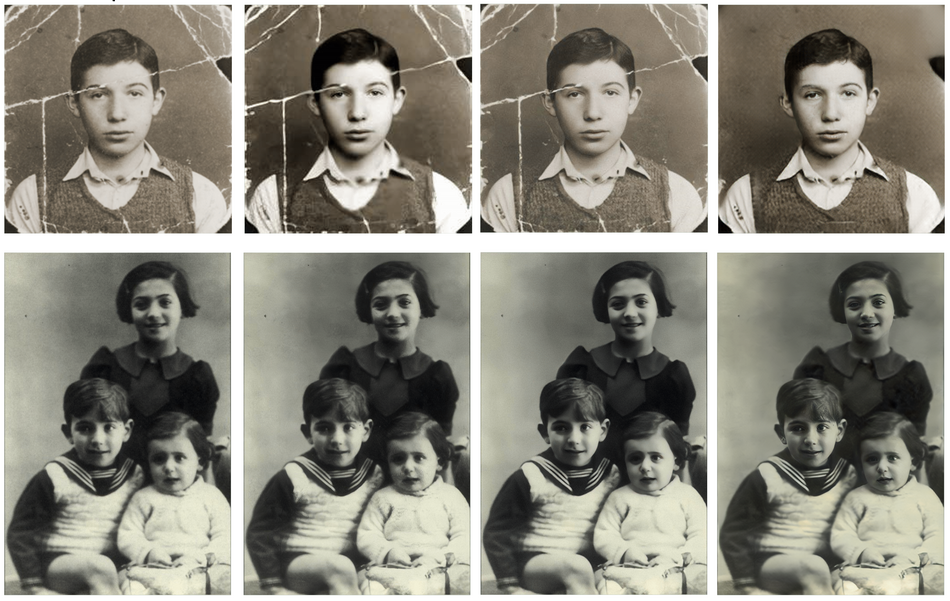
A group of researchers from several universities have joined forces on developing a new method for old image restoration using deep learning.
In their paper, “Old Photo Restoration via Deep Latent Space Translation”, they describe a novel approach for restoring old photos that suffer from severe degradation. The proposed method employs two variational autoencoders (VAEs) in a domain translation framework where the restoration is learned from real images as well as synthetic image pairs.
According to researchers, the main idea here is to close the gap between the two domains in the encoded latent space, as opposed to closing the much larger gap in the image space. They used Variational Autoencoders in order to learn a compact latent representation and be able to successfully close this gap. The first autoencoder network is trained with real and synthetic images, while the second one is trained for clean images. In this way, they tried to learn the restoring of the corrupted images into clean ones.
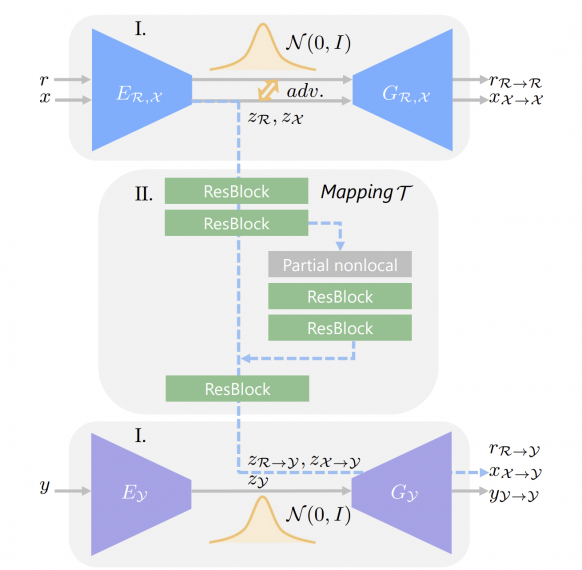
Researchers used the popular Pascal-VOC dataset to create a synthetic dataset of old photos but introducing rendered realistic defects. Additionally, they collected around 5700 old photos to create an original dataset of old images. They compared the proposed method with several baselines among which: Pix2Pix, deep image prior models, CycleGAN, etc.
Results from the experiments showed that the new model is able to successfully restore clean images from old photos. They also showed that the method suffers less from the generalization issue as compared to the baseline methods.
The implementation of the method was open-sourced and can be found on Github. The paper is available on arxiv.