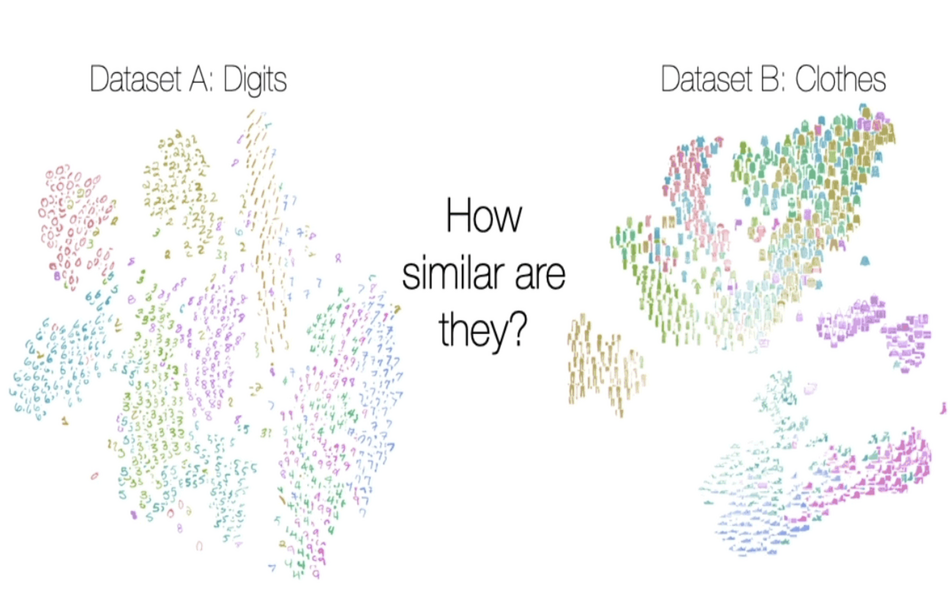
In recent work, researchers from Microsoft have proposed a new method for measuring dataset similarity based on optimal transport (OT) techniques.
Measuring dataset similarity is a non-trivial problem that has been explored very little in past research. On the other hand, having the ability to compare two or more datasets is a very useful feature, especially in the area of Machine Learning, where researchers and practitioners have to deal with dataset choices, dataset combinations and transfer learning.
In their novel paper, named “Geometric Dataset Distances via Optimal Transport”, Microsoft researchers propose what they call OTDD, or a definition for a new metric for comparing datasets. The proposed metric or approach has three significant features: first, it can compare datasets as comparing probability distributions – coming directly from the property of optimal transport methods, second, it can compare datasets regardless of the labels compatibility, and lastly, it can provide a set of (soft) correspondences between individual dataset items.
The OTDD approach, in fact, provides similarity or dissimilarity metrics based on a comparison of two things or two different kinds of probability distributions. The first ones are distributions over dataset labels and the second is distributions over entire datasets. According to researchers, this proposed approach overcomes a number of challenges imposed by the problem of comparing inherently different datasets coming from a variety of sources. Some of these challenges include dataset cardinality differences, label correspondences, and dimensionality differences.

The novel dataset comparison approach can actually provide very useful insights. For example, it can predict transferability (for deciding pre-training strategies), and it can give directions about how to augment one dataset.
More details about the proposed OTDD metric can be read in the paper or in the official blog post.