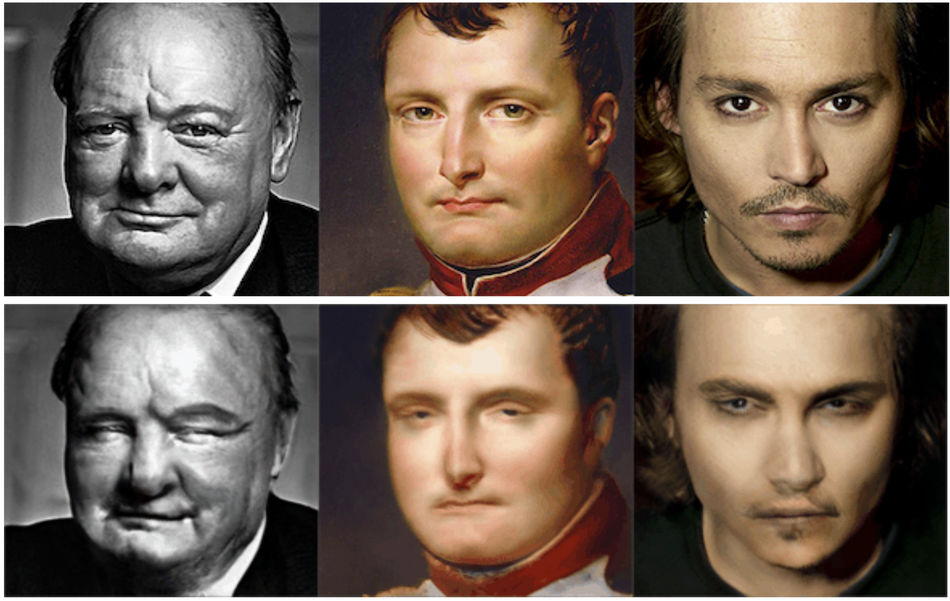
Researchers from Yandex research have proposed a new method for semantic image editing in which a GAN output can be controlled by navigating its parameter space.
The name of the new method – NaviGAN comes exactly from the fact that it allows navigating the visual effects and semantic properties of the generated images. In their paper, Navigating the GAN Parameter Space for Semantic Image Editing, researchers argue that some very useful interpretable directions for image editing exist in the space of generator parameters within a GAN network. Using their proposed method, researchers managed to expand the range of achievable visual effects with a Generative Adversarial Network.
The idea behind their approach is to leverage deep networks in order to discover meaningful directions in the parameter space. More precisely, researchers propose a learning scheme where they take two images – one produced by the original generator and the other by the generator with shifted parameters in some direction. These two images comprise a training sample for a “reconstructor” network which has to predict the direction index and the shift magnitude. In this way, the reconstructor can be trained to predict which shift corresponds to which direction and with what magnitude.
Since the parameter space of Generative Adversarial Network models is usually huge, researchers reduced the dimensionality of their search by constraining the shifts to only particular layers and only particular parameters from the StyleGAN2 demodulation block.
A number of experiments were conducted using 4 popular datasets: FFHQ, LSUN-Horse, LSUN-Church, and LSUN-Cars. The results showed that the method is able to discover meaningful directions in GAN’s parameter space. Researchers conducted all of the experiments using the StyleGAN2 network as one of the most powerful GAN models.
The implementation of NaviGAN was open-sourced. More details about the method can be read in the paper.