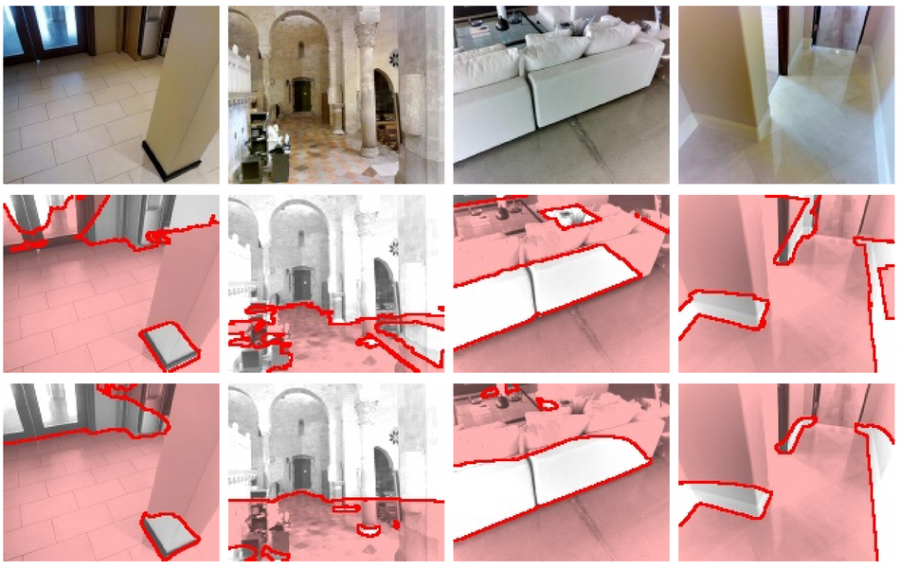
A group of researchers from Niantic and University College London has proposed a new method for free space detection that can predict the geometry of both visible and occluded traversable surfaces.
In the paper, named “Footprints and Free Space from Single Color Image” researchers describe their approach solving the free space detection problem, which is different than most existing approaches. In fact, researchers tackle the problem of traversable surfaces instead of the so-called “free space” in which occlusions were often not taken into account. In the proposed approach the method is able to predict the extent and geometry including hidden walkable (or traversable) surfaces.



Researchers designed a training framework that allows training an image-to-image single deep neural network for free space detection. They used stereo video sequences in combination with information regarding camera poses, per-frame depth and semantic segmentation in a learning framework used to train the free space detection network. Within this framework, researchers define a world model to be given by a four-channel representation: visible segmentation mask, visible depth map, hidden segmentation mask, and a hidden depth map.
The resulting models were trained using KITTI driving dataset, the indoor Matterport dataset and a custom captured stereo footage. Researchers mention that the method performs well on a range of challenging datasets and compares with a number of strong baselines.
More details about the new method can be read in the paper. The implementation was open-sourced and can be found on Github.