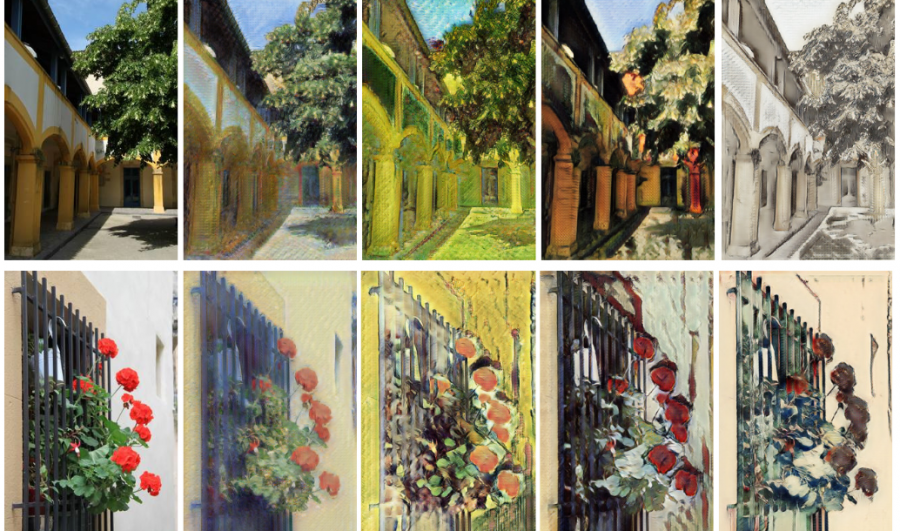
A new method called Gated-GAN was proposed by Xinyuan Chen et al. and makes it possible to train multi-style Generative Adversarial Networks (GANs) for Style Transfer.
A large number of variants of Generative Adversarial Networks or GANs have been proposed in the last few years. GANs proved to be successful in a number of computer vision tasks, and a very popular one is – Style Transfer.
Variational Autoencoders, Generative Adversarial Networks, and other recent generative models were applied successfully to the task of style transfer in images. However, researchers realized that traditional GANs trained for style transfer are only compatible with one style, so a series of (different) models need to be trained to provide users with the choice of multiple styles.
Moreover the well-known issue of Generative Adversarial Networks – the mode collapse results often in unstable training and makes style transfer quality difficult to guarantee. To overcome this problem Xinyuan Chen and his team proposed Gated-GAN – Adversarial Gated Networks for Multi Collection Style Transfer.
The idea is to enable a single GAN model to capture and generate multiple different styles. They propose a network architecture consisting of three modules: an encoder, a gated transformer, and a decoder. The gated module allows different styles to be applied by passing input images through a different branch of the gated transformer.
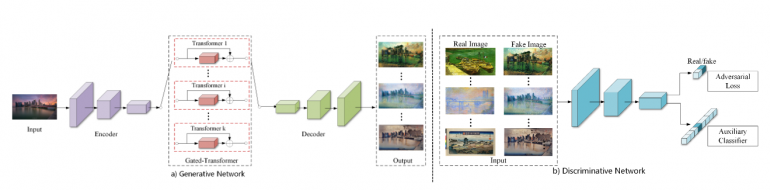
The experiments showed that the proposed model is effective and stable to train. Researchers conducted a large number of experiments to perform a quantitative and qualitative evaluation of the model.
The paper is published as pre-print on arxiv and researchers also released the code implementation of Gated-GAN on Github.