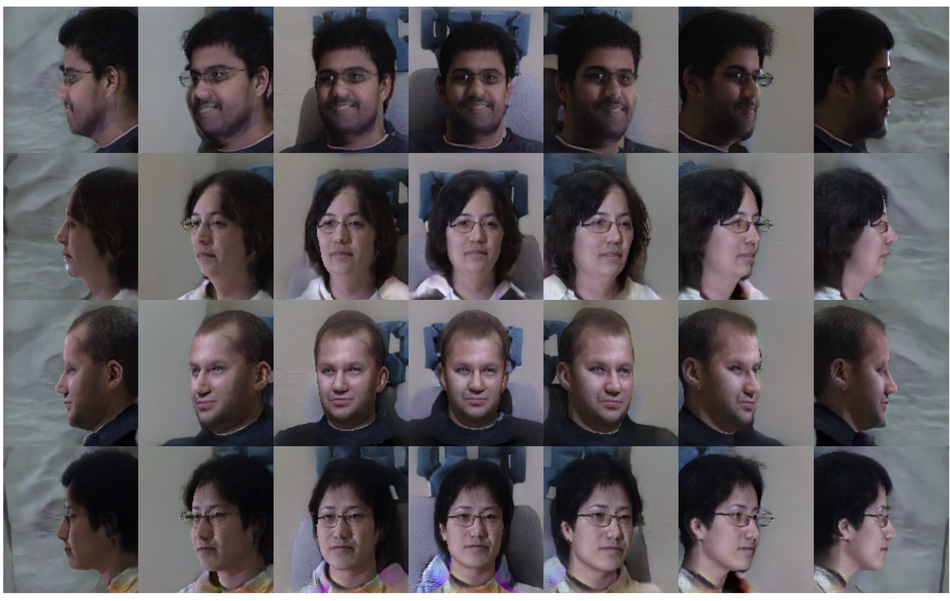
Generative Adversarial Networks are known to be capable of generating highly-realistic synthetic images due to their representation learning capabilities. However, many of the GAN models fail to disentangle identity and pose or facial expression, making it difficult for synthetic data to be used for training facial recognition systems.
In order to overcome this issue, a novel 3D Generative Adversarial Network (GAN) was proposed that can successfully disentangle pose and identity and can consequently generate realistic synthetic data for facial recognition.
The network proposed by researchers from IDEMIA and Ecole Centrale de Lyon, includes a learnable 3D morphable model into its generator and it is able to learn a nonlinear texture model. This generator consists of two CNN networks that learn to generate facial texture and background independently. The facial texture is produced by the CNN from an input given as a random sample of the shape from the 3D model’s learned distribution. Given the texture and the pose as input, the method employs a differentiable renderer with a 3D morphable model which renders the final result without the background. Finally, the background generated by the background generator is included in the rendered image to produce the final result and pass it to the discriminator. The diagram below shows the detailed high-level architecture of the method.
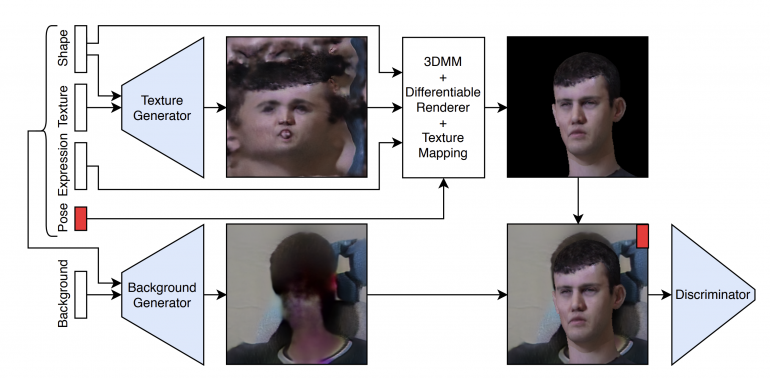
To train the proposed 3D GAN, researchers used the Multi-PIE dataset. They showed that such GAN architecture is capable of disentangling the pose and identity and that it is possible to learn high-quality textures from images with a wide pose range. Researchers then proceeded to use this generator to generate new synthetic identities and perform an experiment in which these identities are used to augment facial recognition model training.
Results from these experiments showed that the new model can be used to improve the accuracy of large-pose facial recognition systems.
More details about the method and the experiments can be read in the paper published in arxiv.