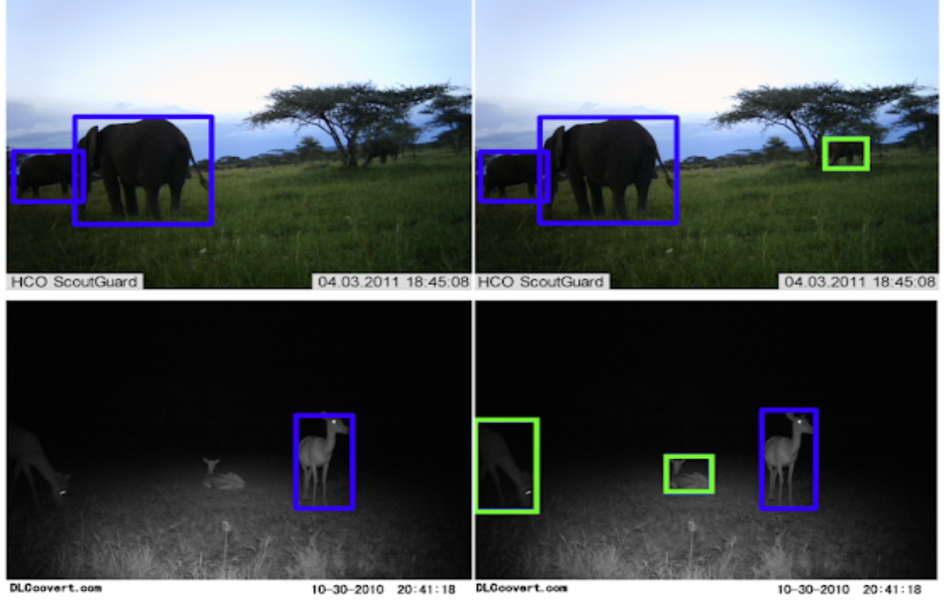
Researchers from Google AI have presented an interesting novel method for object detection that takes into account the image context for improving detection performance.
As part of a large project of ecological monitoring, researchers from both the machine learning and the ecological community have worked together to solve the problem of detecting objects of interest that are out of focus, off-center, or too small. Efforts on collecting expert labeled training data from static cameras placed in nature have been going on for some time, supported by ecological organizations.
However, due to the slow speed of this process, researchers from Google have proposed a complementary approach, by proposing a novel object detection method in their paper ” Context R-CNN: Long Term Temporal Context for Per-Camera Object Detection”. Their method leverages temporal context from unlabeled images in order to improve the performance of the detector. Context R-CNN is an attention-based model that indexes into a long term memory bank constructed from the unlabeled images collected with a specific camera.
The new method was tested on two tasks: detecting animals in camera-trap images and detecting vehicles in driving videos. Researchers tested the Context R-CNN model on the popular Snapshot Serengeti dataset and the evaluations showed that it outperforms state-of-the-art (single frame) baseline by 17.9 % mAP score.
More details about the project can be read in Google AI’s blog post. The paper is available on arxiv.