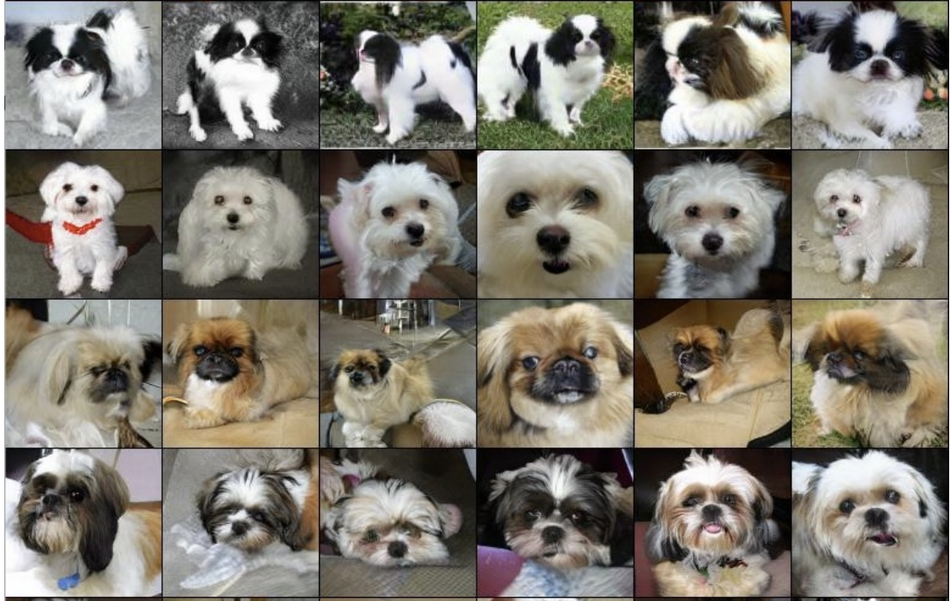
Researchers from Google DeepMind introduced LOGAN – a game-theory motivated algorithm, which improves the state-of-the-art in GAN image generation by over 30%.
LOGAN, which stands for Latent Optimization for Generative Adversarial Networks is a new optimization technique that improves adversarial dynamics in GAN networks.
In their novel paper, researchers argue that optimization of the latent variable z during training can actually improve the dynamics of training Generative Adversarial Network (GAN) models. The new training method that they propose, uses the natural gradient to optimize the latent score at every training iteration. In fact, during forward pass the training procedure remains the same, and the gradient of the loss used to perform a second forward pass. In the second forward pass, this gradient is used to modify (or optimize) the latent variable which is fed as input to the Generator. Then, the gradients of the loss from the second pass are actually used to update the weights of the generator and discriminator.
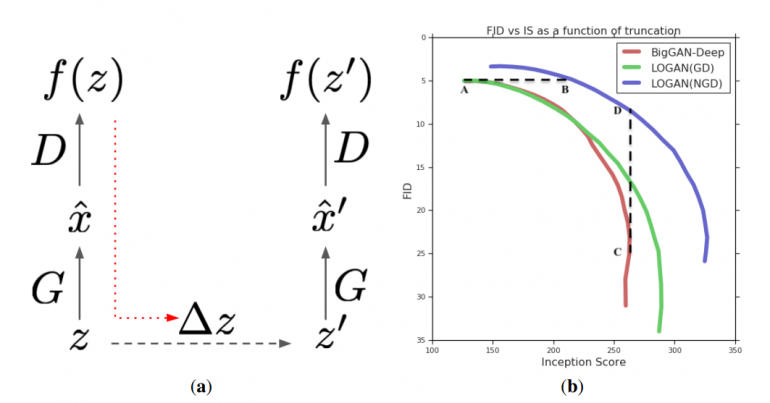
The novel method improves the state-of-the-art results from BigGAN by a large margin while introducing no extra parameters or any architectural modifications. According to researchers, the proposed training method which incorporates latent optimization yields higher quality, more realistic and more diverse generated samples.
To show the performance of the method, they focused on large scale GAN models based on Big-GAN architecture. The model trained with LOGAN achieves an inception score of 148 and a Frechet Inception Distance (FID) score of 3.4 outperforming the current state-of-the-art model by 17 and 32%. The evaluations demonstrated the effectiveness of the method which can actually be applied to any adversarial training task.
More about the proposed method can be read in the official paper published on arxiv.