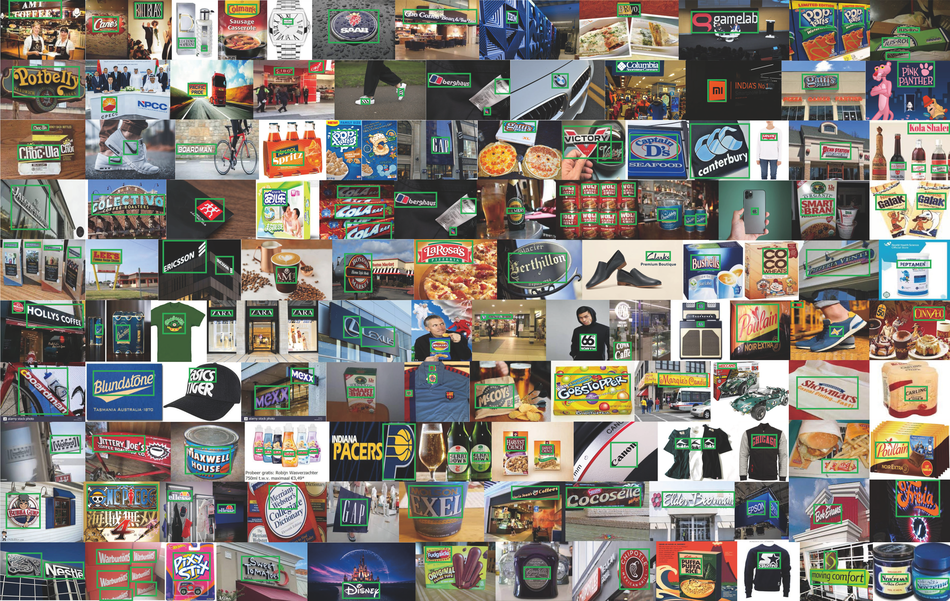
Researchers from Shandong Normal University and the Chinese Academy of Sciences have collected and published a large-scale logo detection dataset.
In the context of branding and brand management, logo detection as such represents an important task with a wide range of applications. Existing logo detection datasets are either small-scale or not diverse enough, and for this reason, researchers decided to collect a larger and more diverse dataset of images for logo detection.
The new dataset, called LogoDet-3K contains 3000 logo categories and over 200 000 manually annotated logos on 158 652 images. It’s variety and comprehensiveness make this novel dataset a more challenging benchmark for logo detection methods.
The collection process was carried out manually and was divided into three phases: image collection, logo image filtering, and logo object annotation. Researchers managed to collect a large-scale dataset and they extended the popular YOLOv3 object detection model to make it suitable for the task of logo object detection. In fact, they incorporated focal and CIoU loss into the YOLO training framework to tackle the problem at hand.
According to the results that researchers reported the new Logo-Yolo model achieves a 4% improvement over existing models and YOLOv3 trained on the newly collected dataset LogoDet-3K. The novel dataset for logo object detection was open-sourced and can be found here. More details about the data collection process and the logo detection models can be found in the paper published on arxiv.