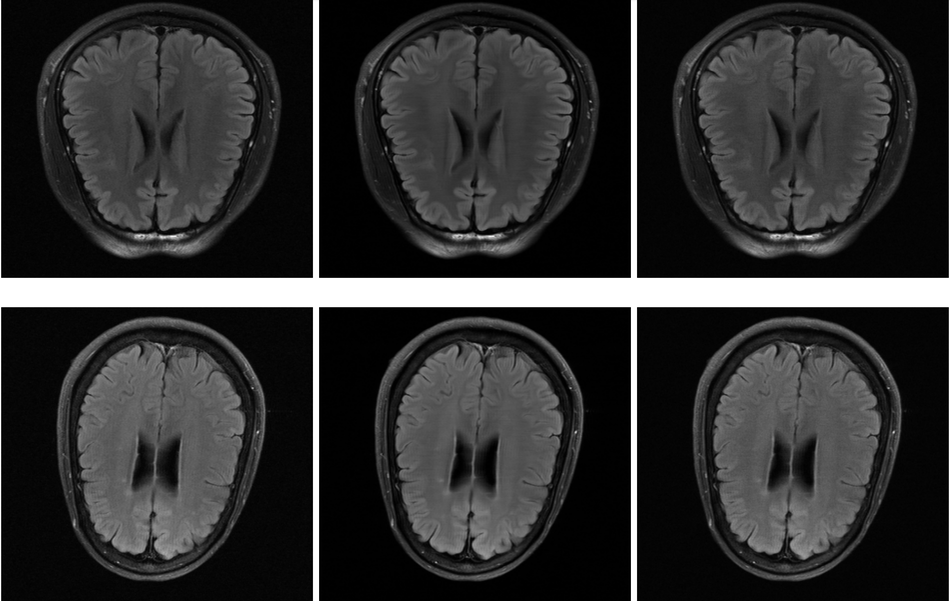
In a 2-year-old collaboration project, researchers from Facebook AI and New York University Langone Health, have developed a new way to accelerate MRI scanning procedures by leveraging AI technologies.
The initiative, called FastMRI aims to use AI and Computer Vision towards speeding up the MRI scanning and creating accurate MRI scans with much fewer data required. The group has published a series of papers during its 2 years of project development and has built upon previous research in the field of MRI scan acceleration.
In the latest paper in this series, researchers proposed a very powerful end-to-end method for reconstructing undersampled multi-image data from MRI scans obtained using parallel imaging and compression. The method uses Variational Autoencoder Networks in a multi-stage learning framework, where a k-space input scan is reconstructed by minimizing a structural similarity loss function (SSIM).
The network, called E2E-VN applies a number of refinement steps to the vector of multi-coil k-space data. It consists of several larger modules (or components): a Data Consistency module (DC), a Refinement (R) module, and a Sensitivity Map Estimation module (SME). The full architecture is depicted in the diagram below.
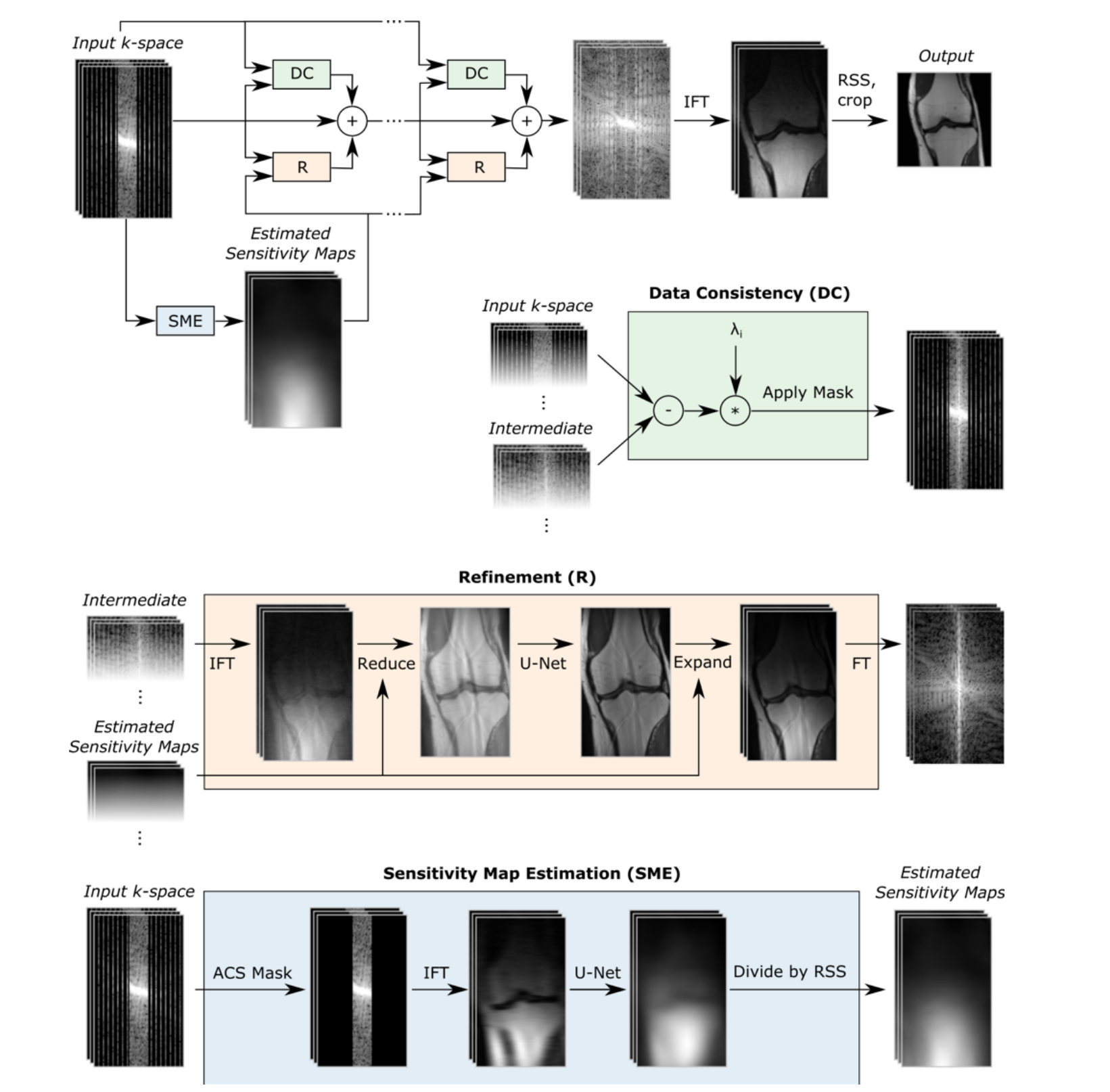
The proposed method was evaluated using the fastMRI dataset and was compared to several other architectures: Variational Networks, Variational Networks with U-Net (VNU), etc. Results showed that the E2E-VN network achieves state-of-the-art results for both brain and knee scans from the fastMRI dataset.
The implementation of the method is open-sourced and can be found on Github. The paper, along with the other papers from the FastMRI project can be accessed here.