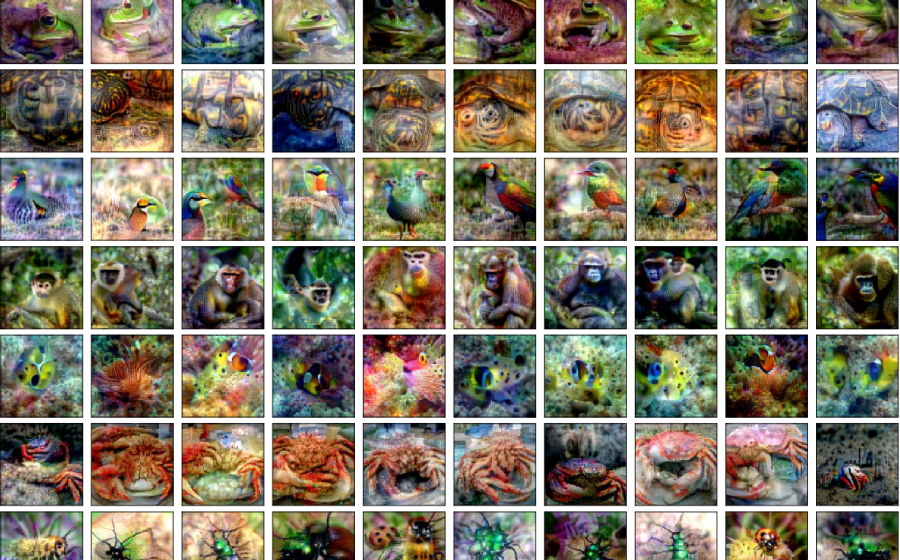
Researchers from MIT have shown how a single classifier model can be used to tackle multiple different computer vision tasks. In their novel paper, researchers explore the potential of robust classifiers towards applications in challenging computer vision tasks.
The idea behind this approach is to exploit the robustness of classifiers (deep neural network models) and address different problems without designing problem-specific architectures. Researchers propose to use predicted class scores in an optimization problem to solve tasks such as image generation, image inpainting, image-to-image translation, super-resolution, and interactive image manipulation.
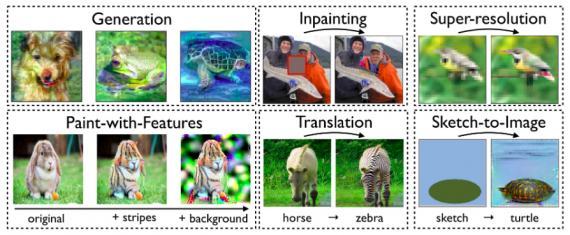
They observed that maximizing the loss of robust classifier models leads to realistic instances of other classes. Leveraging this fact, they were able to solve multiple complex computer vision tasks using a single classifier. Researchers built a versatile computer vision toolkit based on this.
The performance of the proposed approach was shown in the defined computer vision tasks. Researchers mention that they refrain from extensive tuning and task-specific optimizations in their experiments to emphasize the potential of the proposed idea.
The implementation of the proposed method was open-sourced and is available here. More details about the method and the obtained results can be found in the official paper.