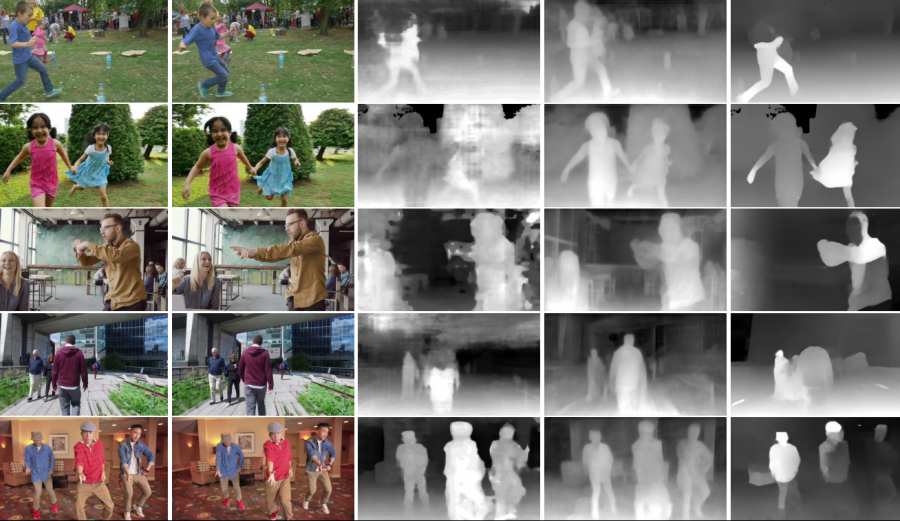
Researchers from Google AI have proposed a new method for depth estimation of moving people. The new method is capable of handling videos where both the people and the camera are moving.
As a particularly challenging problem, depth prediction of moving objects was not explored much in the past. Depth estimation methods, generally filter out dynamic objects in scenes or simply ignore them and therefore an incomplete depth map is obtained containing only background and static objects.
In this approach, researchers took advantage of the popular “Mannequin Challenge”, where people all over the world were uploading videos of imitating mannequins by freezing in some natural pose. The fact that in this kind of videos (uploaded by users on Youtube) the camera is moving around all static objects allowed researchers to construct a large dataset of depth maps corresponding to the videos.
For the task of acquiring accurate depth maps as labels, they used triangulation-based methods such as multi-view-stereo(MVS) and collected a dataset of around 200 videos. The dataset is of high variability since the videos are taken from the Mannequin Challenge, where people tried to naturally pose in a wide variety of everyday situations.
Researchers proposed a neural network model for depth estimation trained in a supervised manner using the collected Mannequin Challenge dataset. They frame the problem as a regression problem where the input to the network is the RGB frame, depth mask of the background and binary mask of the people in the image. According to them, the depth from parallax (depth mask of the background) will give strong depth cues and help to solve the problem in a more efficient manner. This depth mask is computed by computing the 2D optical flow between two frames.
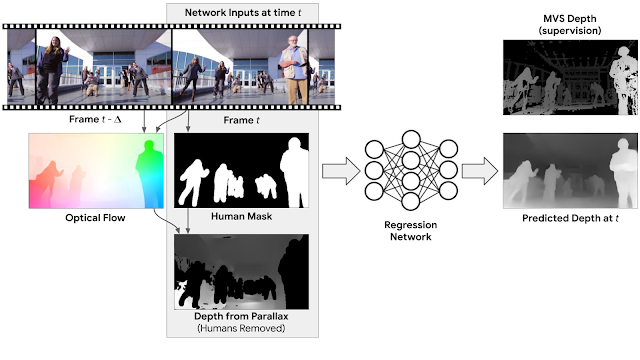
So, the task that the network is solving is basically “inpainting” the depth values for the masked regions with people. Results show that the network is able to learn depth estimation of moving people and researchers mention that it can generalize and handle any natural video with arbitrary human motion.