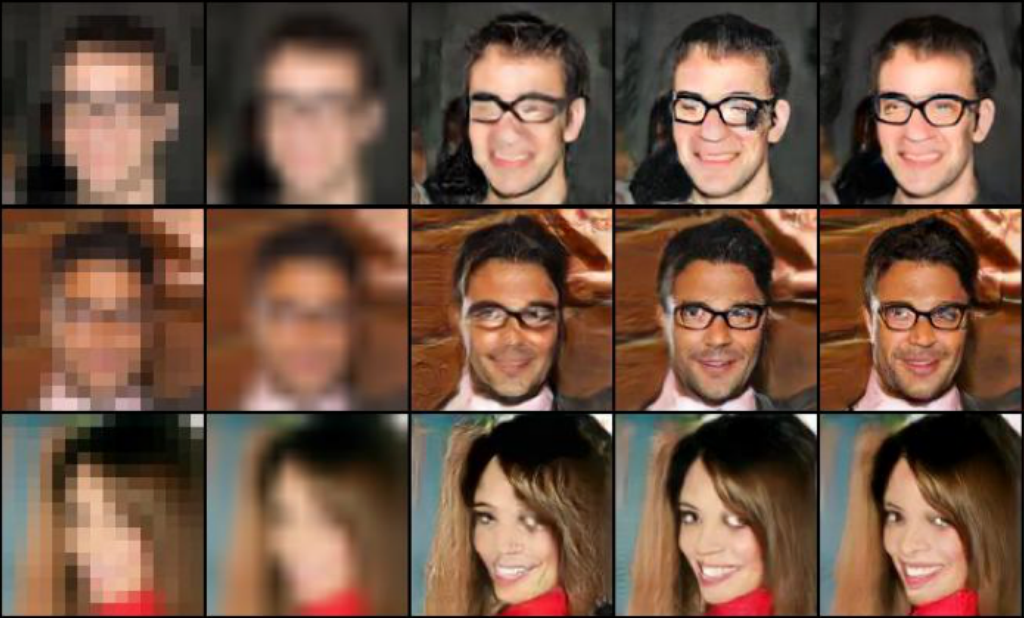
Researchers from the Korean Advanced Institute of Science and Technology, have developed a method for face Super-Resolution that can generate photo-realistic 8x super-resolved images.
The new method is based on the GAN architecture where a generator is trained to generate realistic images. Researchers proposed several improvements and novel techniques in order to obtain a method that will be able to do super-resolution of even very small images.
First, they employed progressive training method which splits the network into successive steps and each of them produces output that is with progressively higher resolution. Second, they proposed a novel facial attention loss and this allows the network to focus on restoring richer facial attributes. Last, they compressed the state-of-the-art facial alignment network (FAN) into a smaller network to reduce computational cost and make it more suitable for the task of facial super-resolution. The architecture of the proposed method can be seen in the diagram below.
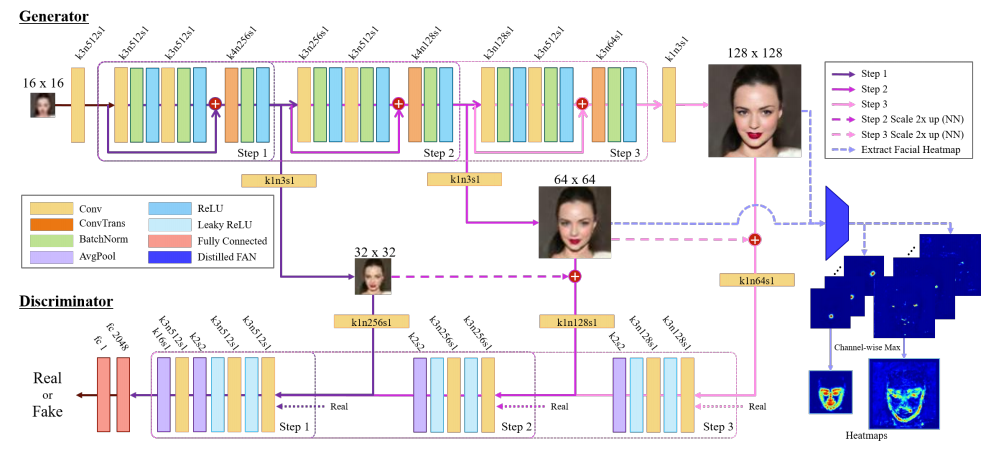
The proposed method was tested on two datasets: CelebA and AFLW, which researchers split into aligned and not aligned. The evaluations showed that the method outperforms current state-of-the-art methods for facial super-resolution. Researchers also quantified the improvements in image quality across the three proposed ideas: progressive training, the novel loss function and the distillation of the FAN network. They mention that their method is able to produce visually plausible results which contain accurate facial details.
More about the method can be read in the paper published on arxiv.