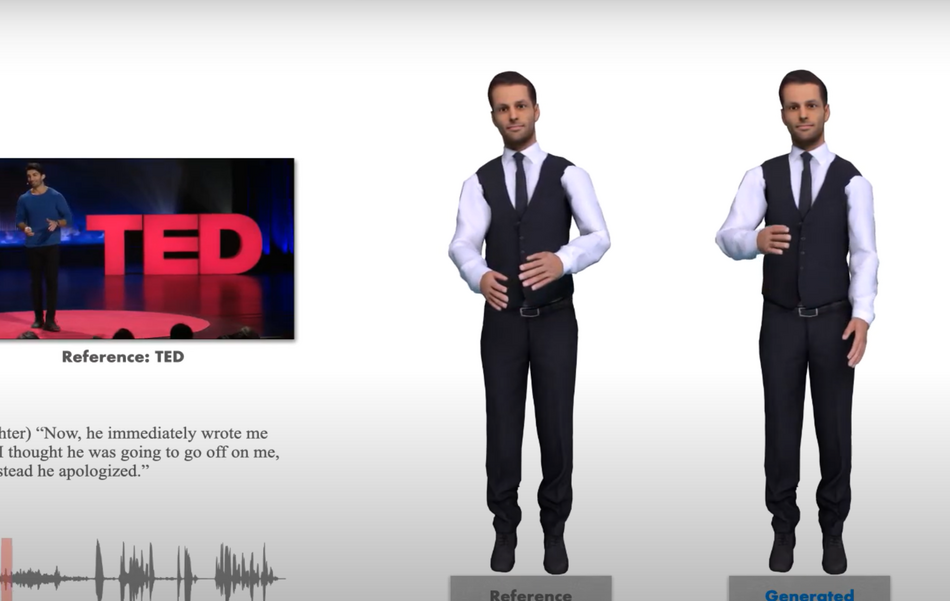
A group of researchers from the University of Science and Technology, ETRI from Korea have developed a method for learning speech gesture generation using online speech videos.
In their latest work, researchers have proposed an automatic gesture generation model that extracts information from a multi-modal context (speech, speaker identity, text) as opposed to data-driven methods that try to learn the gesticulations directly from video data. Within their adversarial training scheme, they try to learn realistic, human-like gestures and movements that correspond to the speech and its rhythm.
The proposed model uses three data modalities that are directly captured from an input speech video: the speech audio, the transcribed text, and the speaker’s identity. Different encoders are used for speech and text respectively to obtain an intermediate representation which together with a “gesture style” sampled from a style vector, goes into the Co-speech Gesture Generator model. Researchers used Huber and adversarial loss to train the model. The data used for training was the large scale TED gesture dataset that contains speech data from various speakers, enhanced with an additional 471 TED videos. The total size of the training dataset was 1766 videos.
Researchers conducted a number of experiments to evaluate the method both quantitatively and qualitatively. They also performed a user study to evaluate and compare the proposed method with existing methods. Results showed that the method outperforms existing methods in several aspects: human preference, human-likeliness of the motion and speech-gesture matching.
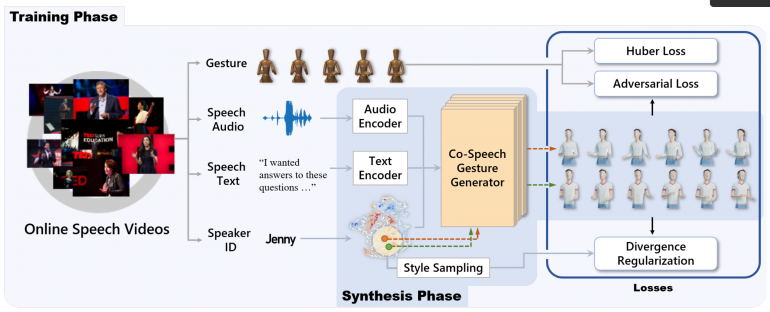
More about the method can be read in the paper published on arxiv. The implementation was open-sourced and it is available on Github.