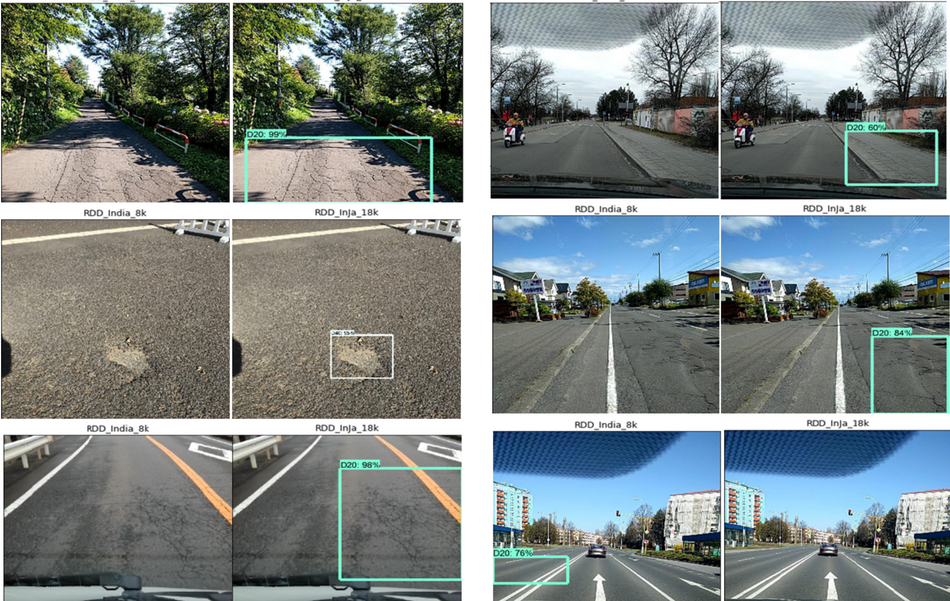
A group of researchers from several research institutes and universities has collaborated together on a project to develop a road damage detection model that works well in multiple countries.
Considering the limitations of previous research done within the topic of road damage detection, researchers have started the project where they wanted to build a more robust model for automatic road damage detection. As part of this project, they assessed the usability of a previously developed smartphone-based method (a Japanese model) for road damage detection in other countries. Furthermore, they collected a large-scale image dataset consisting of more than 26 000 images from multiple countries around the world.
Using the novel dataset, in combination with existing datasets for road damage detection, researchers developed a more robust road damage detection models which perform satisfactorily in multiple countries. They divided the models into two groups: Single Source Models (trained on one source of data, and tested on heterogeneous data), Multiple Source Models (same source-same target models and trained with combined data from multiple countries).
The experiments were performed separately for each target country (Japan, India, the Czech Republic). Researchers concluded that for single-source models it was difficult to perform well on out-of-distribution data (the different target countries) irrespective of the amount of training data. For the multiple source models, they mention that results vary depending on the target country.
The collected dataset as part of this research was published and it is available on Github. More about the models and experiments can be read in the paper.