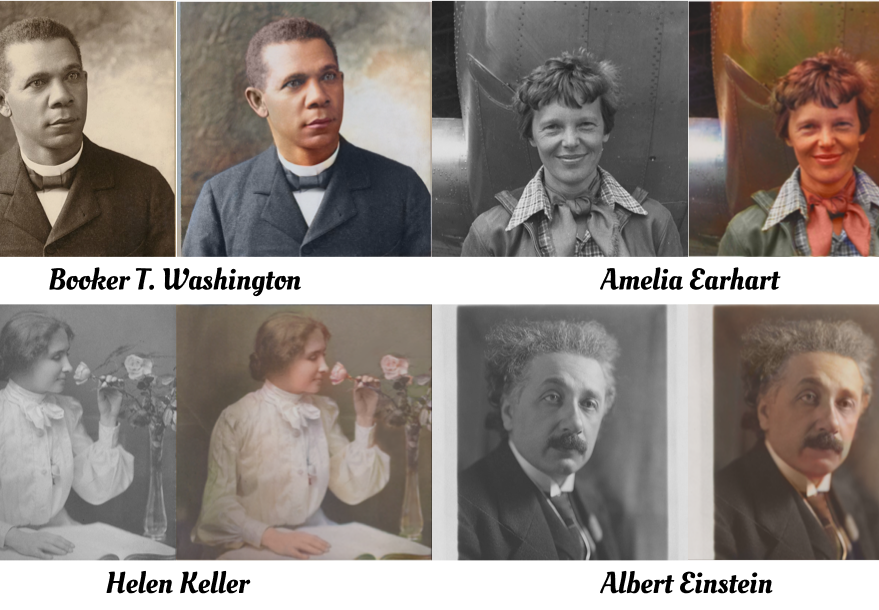
The pix2pix neural network was trained to automatically color old black-and-white photos. The model will allow colorization specialists to reduce the time spent on color selection and manual coloring of images.
In addition to manually coloring the original black-and-white photo, the colorization process is accompanied by extensive research on similar color images and historical data required to accurately restore colors. The conditional generative-adversarial neural network pix2pix allowed to simplify this process. The model consists of two parts (Fig. 1): a generator trained to color black-and-white images, and a discriminator. For training, a dataset consisting of color images was used. By removing the color from each image, pairs of color and black-and-white images were generated. The task of the generator was to color the black and white images so as to make the discriminator think that these are originally colored images, and so that the original colors are restored as accurately as possible. The discriminator is trained to distinguish the original color photos from those colored by the generator. Thanks to this competing process, the model begins to color the images more reliably with each iteration.
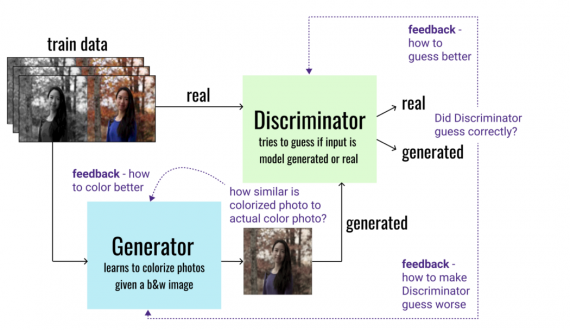
The neural network was trained in two stages. In the first stage, the COCO dataset, consisting of 35,000 object images, was used to train the neural network on the typical prevalence of colors in the environment. In the second stage, a dataset of 2,000 portraits taken from Unsplash was used to train the model to color people’s faces. Since older photos are more blurry than digital photos, a random blur was added to each image from the datasets. The results of the restoration are shown in Figure 2 together with the manually adjusted colors. Despite the drawbacks of the restoration visible in Figure 2, namely, the coloring of some parts of the image in too bright colors, the neural network correctly recognizes the colors in the entire image. This reduces the time spent by colorists on color matching and manual coloring, reducing their work to a quick color correction. A special feature of the model is that there is no need to recognize objects in the image-a method used in most state-of-the-art approaches to image coloring and requires large marked-up datasets.
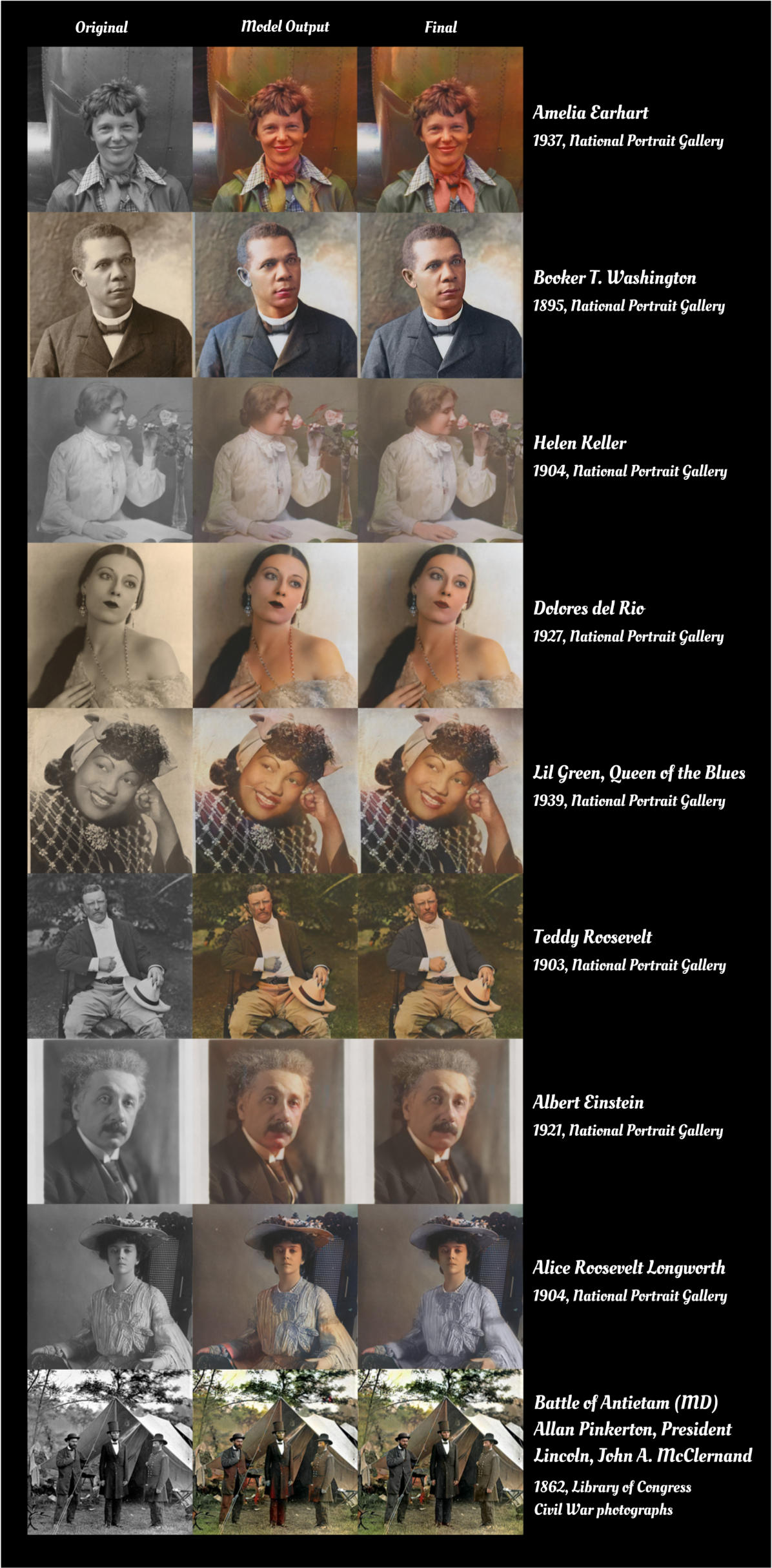