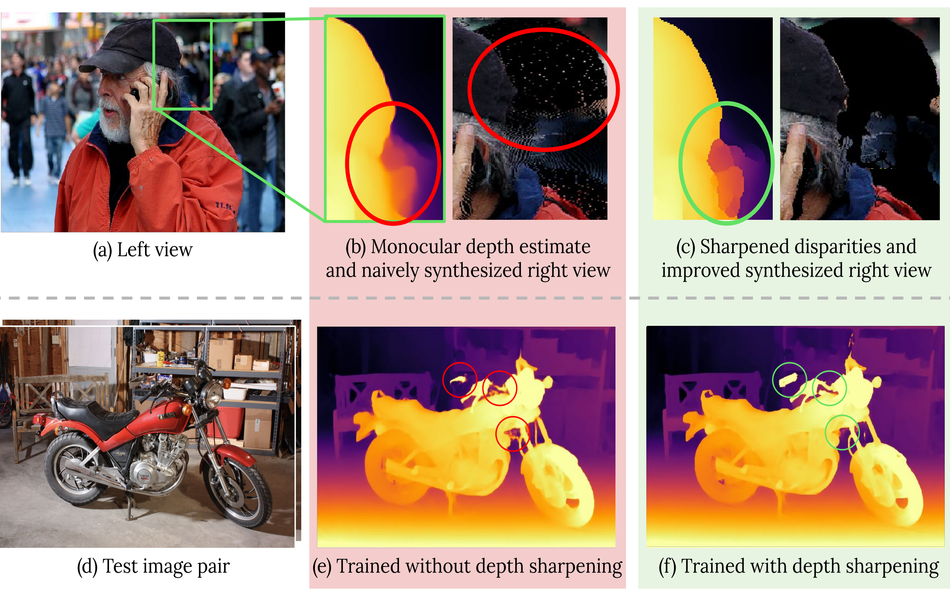
A group of researchers from Niantic, the University of Edinburgh and UCL has developed an interesting method that learns to generate stereo images from a single monocular image.
In their paper, named “Learning Stereo from Single Images”, researchers explore the idea of generating stereo image pairs as training data using monocular images and a monocular depth estimation model. They argue that typically, stereo-based methods are trained using synthetic data when real stereo data is not abundant, and consequently this affects the performance of these methods, especially when deployed in real systems. To overcome this problem, researchers designed a learning pipeline which uses “flawed” disparity maps obtained from monocular depth estimation models, to produce realistic training stereo pairs.
The proposed pipeline starts by converting the input image to an estimated depth map using a deep neural network, which is converted to a disparity map. This disparity map is further refined by sharpening before it is being passed to the forward warping stage. Lastly, after the forward warping, background filling is the last step that generates the corresponding ‘right’ stereo pair image.
Experiments were conducted using several popular benchmark datasets. Researchers showed that the method outperforms stereo networks trained with synthetic data, evaluated on KITTI, ETH3D, and Middlebury.
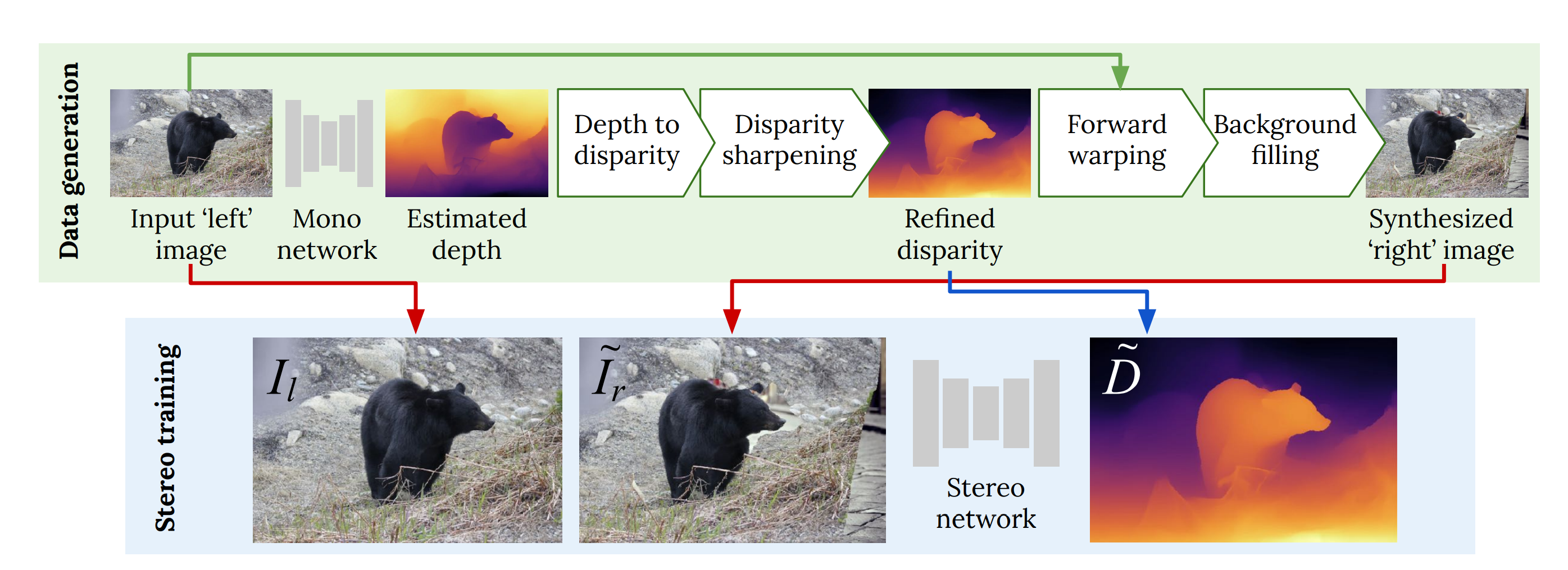
More in detail about the proposed method, the conducted experiments and results can be read in the paper published on arxiv. The implementation was open-sourced and is available on Github.