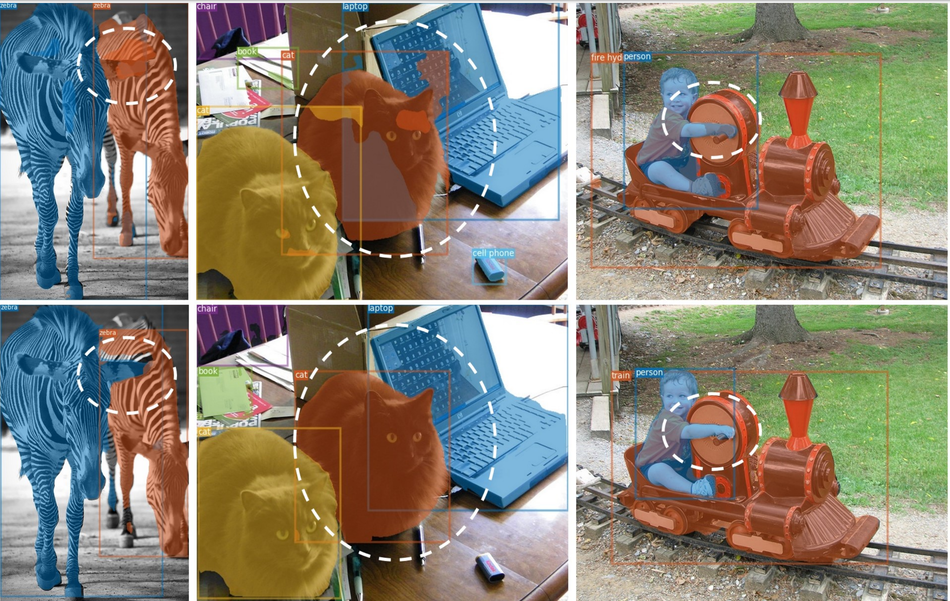
A group of researchers has proposed a new instance segmentation method that achieves state-of-the-art performance on several popular benchmark datasets.
Researchers’ main contribution is a novel spatial preservation module (SP) which plays the role of feature pooling mechanism in the proposed single-stage segmentation method. The idea behind this module is to preserve as much spatial information as possible from one object instance. The segmentation method was built upon the previous FCOS detection method. In fact, the two standard classification and regression branches were replaced with mask-specialized classification and regression branches for the purpose of instance segmentation. The classification branch predicts classification scores and assigns spatial coefficients to regions of the bounding boxes of an instance. In the end, these coefficients are used by the newly proposed SP module to generate separate mask predictions for separate regions/parts of a bounding box.
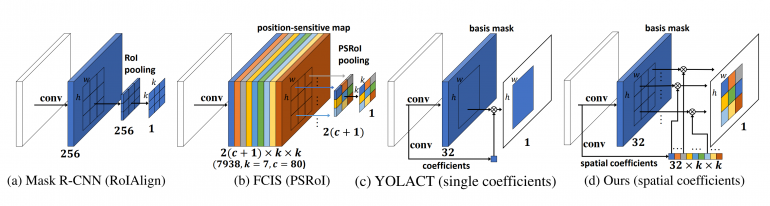
The method was evaluated on the wide-known COCO benchmark. In the paper, researchers mention that SipMask outperforms the current state-of-the-art single-stage method. Moreover, the new method provides a four-fold speedup over existing state-of-the-art segmentation methods.
The implementation of SipMask was open-sourced and it is available on Github. More details about the method, the experiments and results can be found in the paper published on Arxiv.