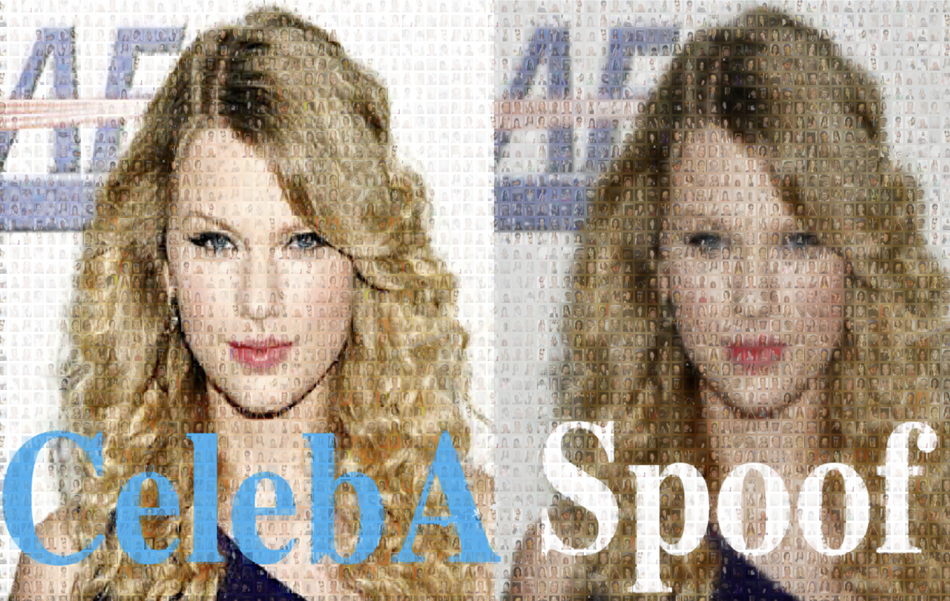
A group of researchers has collected and released a novel anti-spoofing dataset built on top of the popular CelebA dataset.
The novel dataset, named CelebA-Spoof is a large scale dataset containing more than 625 000 images of more than 10 000 subject participants. The dataset was collected with the intention to build a large and diverse dataset for anti-spoofing considering the current limitations (in both size and diversity) of existing datasets for this purpose.
In the paper, researchers mention the three key improvements that CelebA-Spoof will introduce over other datasets. Quantity or size, diversity, and annotation richness are the key features of the new dataset which make this dataset an effective training data source for face anti-spoofing methods. Part of the annotations were borrowed or inherited from the original CelebA dataset, while researchers introduced 10 new types of annotations that capture spoofing types.
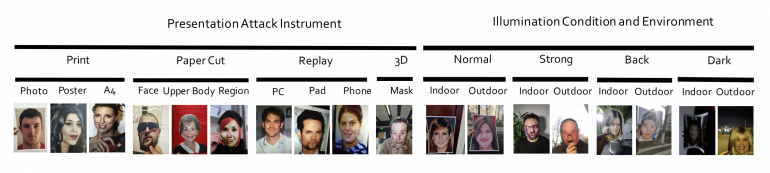
The additional annotations were done by 8 collectors which were hired to carefully collect spoof data. The data collection process was designed specifically in order for the dataset to offer the needed diversity. Spoofing types were categorized in a hierarchy which first classifies the types into 4 groups: print, paper-cut, replay, 3D. A similar hierarchy was designed to also capture various environmental conditions. The scheme can be seen in the image below.
Researchers showed that CelebA-Spoof is effective by training existing models that achieve state-of-the-art performance on several standard benchmarks. The dataset was released under a restricted license which allows dataset use for non-commercial and educational purposes.