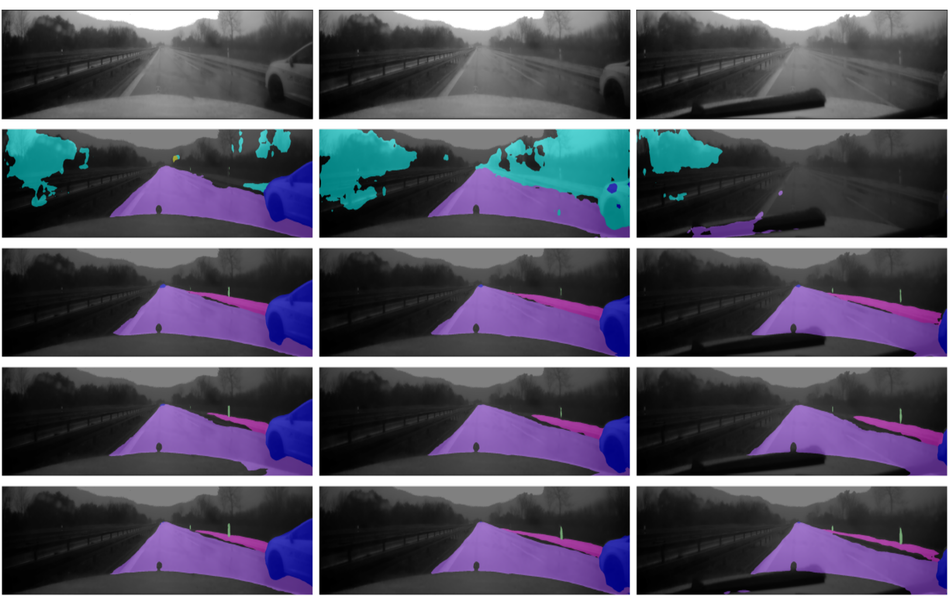
Researchers from the University of Ulm have proposed a segmentation model that can perform well in adverse weather conditions.
Their proposed method builds upon previous research in the direction of using recurrent neural networks for video segmentation. Past research has shown that single-image segmentation as such fails to produce good results in bad weather conditions, and the alternative solution for this is to use a temporal model i.e. video segmentation. However, the computational cost of doing video segmentation as opposed to single-image segmentation is much higher and therefore it makes this approach not suitable especially for real-time applications.
In order to overcome these problems, researchers proposed a variant of the recurrent units in LSTM-ICNet, a model that performs well on video segmentation. The proposed change makes the model more light-weight and thus speeds up the inference by 23 percent while maintaining the same level of segmentation performance.
The modification refers to the building blocks of the network wherein the recurrent unit additional branch was introduced with preceding two 1×1 convolutional layers (as illustrated in the image below). This change solely introduces significant speedup in the model inference.
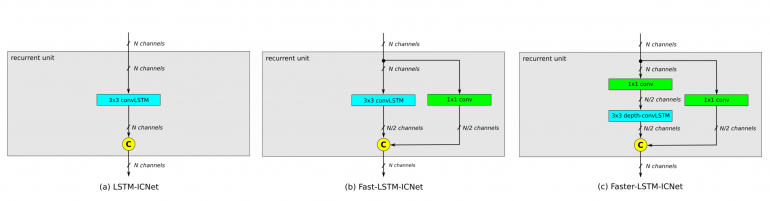
Researchers evaluated the model both quantitatively and qualitatively on two datasets: Cityscapes and AtUlm-Dataset. The results confirmed the superior performance in terms of the inference speed of the new model over existing baselines.
The implementation of the network was open-sourced and can be found on Github.