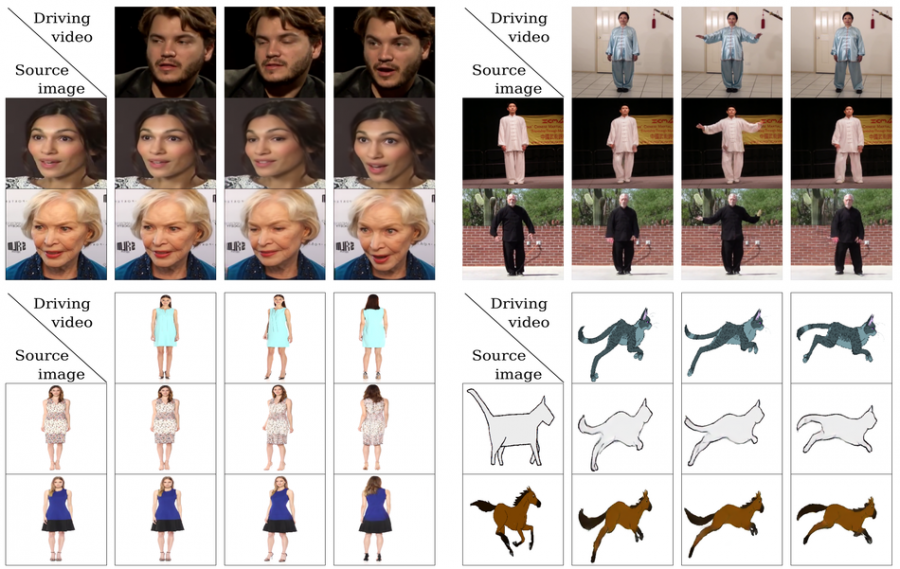
A group of researchers from the University of Trento has proposed a new state-of-the-art method for highly-realistic image animation.
The problem of image animation has gained more and more attention during the past years, and along with that a large number of applications and use-cases for image animation models have appeared. Researchers led by Aliaksandr Siarohin have designed a new self-supervised learning framework for learning an image motion model from data.

Within this framework, the motion and appearance information is decoupled and learned in a completely self-supervised manner. The proposed method works by detecting keypoints together with local affine transformations which are used to define a motion representation altogether. This representation is learned in a fully unsupervised manner from a source frame using a “keypoint detector” network. Besides the source frame, the method takes another, so-called “driving frame” as input. A dense motion network consumes the source image together with the motion representation to generate a dense optical flow from the “driving” frame to the source frame. In the last stage of the proposed method, a generator module takes the source image and the dense optical flow to render a target image.
The proposed method was trained and evaluated using 4 different datasets: VoxCeleb, UvA Nemo, BAIR robot pushing, and a custom dataset collected from tai-chi videos from YouTube. Results showed that the method outperforms state-of-the-art methods on all benchmarks.
Researchers open-sourced the implementation of the new method and it can be found here. The paper was published in the proceedings of NIPS.