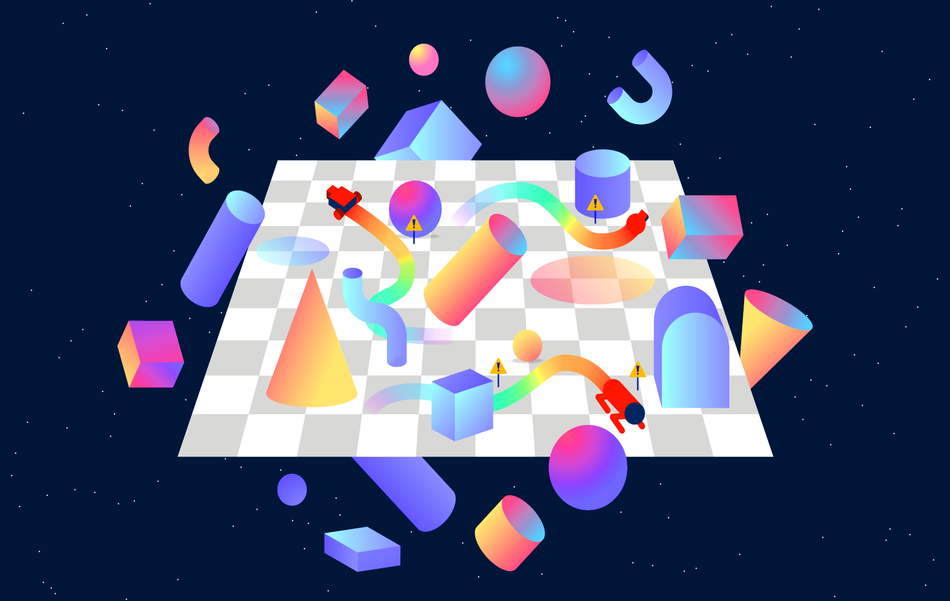
OpenAI has announced the release of SafetyGym – a set of environments and tools for testing reinforcement learning agents towards safety constraints during training.
Considering the deployment and real-world applications of reinforcement learning, researchers from OpenAI decided to build a common testing ground where agents can be evaluated in terms of their costly mistakes, both when performing a task and also while learning.
SafetyGym helps evaluate and study the performance of Constrained Reinforcement Learning, where agents have to follow safety constraints. According to OpenAI, the new suite of RL environments provides richer environments with widened difficulty and complexity.
All of the environments which are part of SafetyGym are designed to be cluttered and a robot has to learn how to navigate through them and achieve it’s given task. There are three types of robots: Point, Car, and Doggo, three main tasks (Goal, Button, and Push), and two levels of difficulty for each task in each of the environments. Whenever a robot does something that violates safety – for example running into a clutter, it provokes a cost in addition to the task reward. In this way, the agent is penalized and evaluated in terms of safety constraints.
OpenAI researchers used SafetyGym to evaluate standard reinforcement learning algorithms such as: PPO, TRPO, Lagrangian penalized versions of PPO and TRPO, and Constrained Policy Optimization (CPO). They mention that current approaches for reinforcement learning and constrained reinforcement learning can easily solve the simplest environments of SafetyGym, while for the hardest and more challenging environments are too difficult for these agents.
SafetyGym is available on the following link. More details about the implementation and the benchmarking of current RL approaches in the new environment can be found in the paper, or in the OpenAI’s blog post.