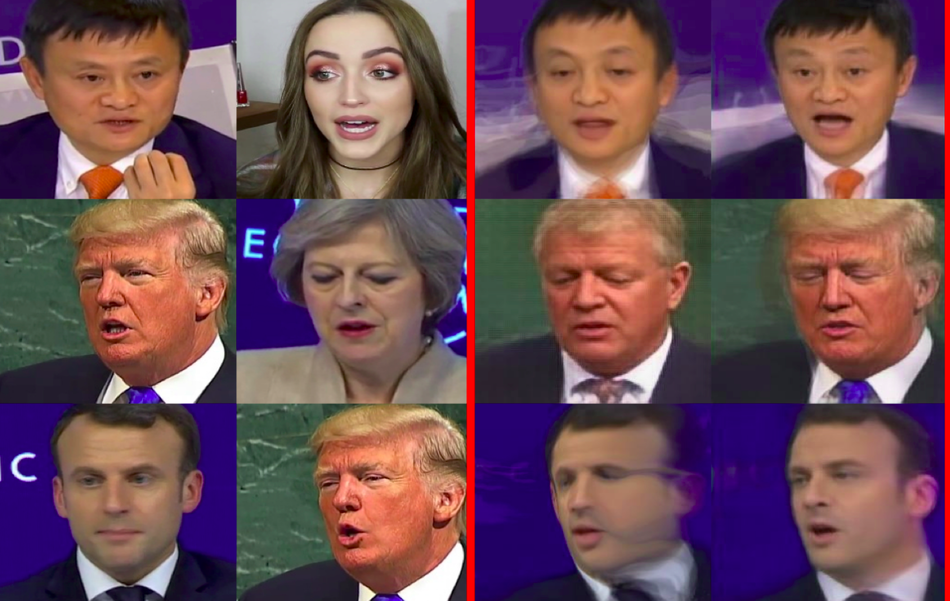
A group of researchers from Hyperconnect has proposed MarioNETte – a neural network model that changes the facial expression of a person in an image.
Given an input image and a target facial expression (as well given as image), MarioNETte produces a realistic novel image where the person has the target facial expression and preserved identity. The method is based on the paradigm of Generative Adversarial Networks where a conditional generator generates a reenacted face and a discriminator predicts whether the image is real or not.
Researchers proposed a generator that consists of several components: a pre-processor, driver encoder, target encoder, blender, and a decoder. The preprocessor takes the input image and also the target image with the target facial expression (named driver in the paper) and uses a 3D landmark detector to extract facial keypoints. These keypoints are passed to the driver encoder and the target encoder for the driver and the target images, respectively. The blender component takes the driver encoded feature map and also the target feature maps to generate a mixed feature map, which later the decoder uses to synthesize the final output image.
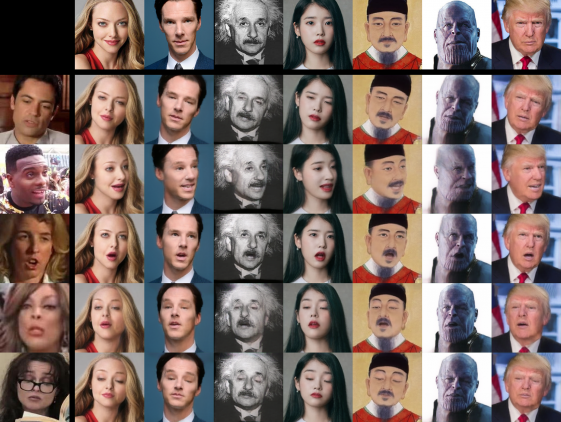
The proposed architecture was evaluated using the VoxCeleb1 dataset. The experiments showed that MarioNETte outperforms baselines and current state-of-the-art approaches for face reenactment using few-shot learning. The outputs from the method are highly realistic images where the identity of the target person is well preserved.
More details about the method and the evaluations can be found in the project’s website or in the paper published on arxiv.